AI hype vs reality: AI in the real world
This post explores the current state of AI, contrasting the widespread hype with real-world applications that deliver tangible business value. It highlights the overpromising nature of generative AI and emphasizes the immediate benefits of predictive AI, urging companies to adopt a targeted and responsible approach to AI implementation.
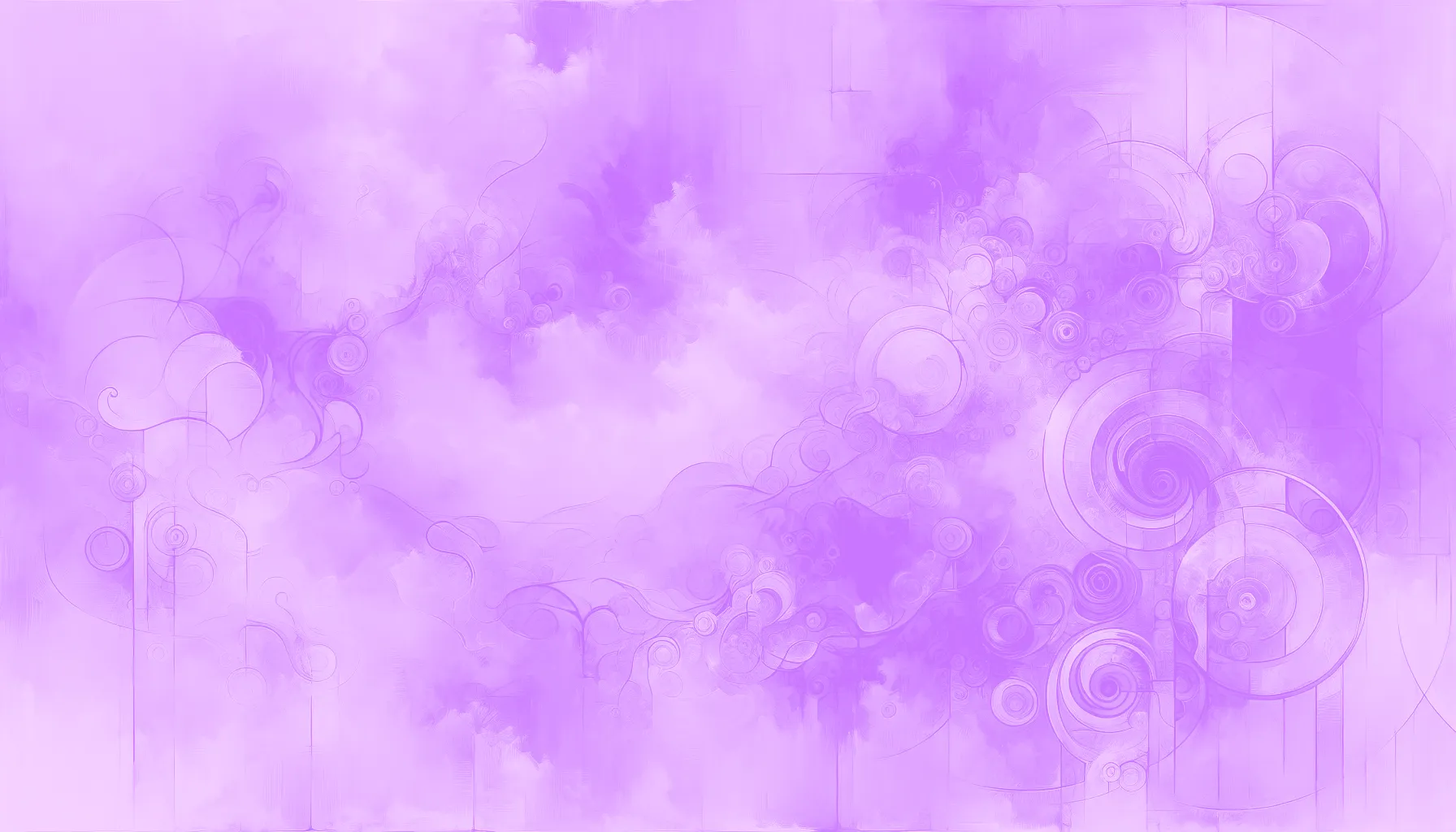
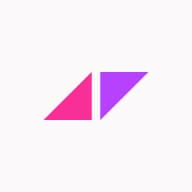
Is the artificial intelligence hype cycle approaching the inevitable trough of disillusionment, as hype and reality collide head-on? Is the AI bubble, much like the internet bubble of years past, about to burst?
AI is moving at a frantic pace, and with its advancement comes a massive amount of investment, which has led to inevitable market saturation and a lot of inflated expectations. As of the summer of 2023, Gartner had already identified that generative AI was reaching the 'peak of inflated expectations' and was about to enter the dreaded 'trough of disillusionment'.
Even the ever-promoting, all-is-well tech titans are starting to sound the alarm on overpromising about the power of AI. Recently both Amazon and Google have reportedly been telling their sales teams to tone down on hyping AI too much to customers. Today's AI leaders are concerned that they are overpromising, and underdelivering.
But all is not hype when it comes to AI, there are many real-world business applications where AI is making a big difference and delivering true value to both the business and its customers. Accordingly, Gartner expects 2024 to be the year where the companies that successfully deploy AI do so through a more targeted and disciplined approach.
So, what is AI hype and what is AI reality? And how are people using AI successfully in the real world to make a measurable difference to a business's bottom line?
AI hype
The amount of AI hype is extraordinary, even in the world of technology hype. Many of us who have been around the block a few times have heard this story before. An amazing new technology arrives that will change the world, and then companies hype the technology endlessly, leading to market saturation, end-user fatigue, and missed expectations. Eventually, the technology makes its way into mainstream adoption, but typically through more distinct use cases and targeted real-world applications. This process is what Gartner's Hype Cycle attempts to represent graphically.
What is AI hype? When you go to a technology conference and an AI futurist talks in vague generalities about how AI will solve every future problem while giving no specific use cases. Or when omnipresent tech CEOs recycle their chatbot marketing from 30 years ago, put an AI in front of it, and tell you your AI assistant will help you in every conceivable way, like a virtual butler. This is AI hype.
For the past year or so, the AI hype discussion has been dominated by generative AI. And while some demos of generative AI were promising, their real-world application at this time has been oversold somewhat to the general public and business world.
AI reality
While up to now generative AI has gotten much of the attention, predictive AI has become the hot topic of conversation in business circles for delivering immediate business value in challenging economic times. Predictive AI can work in tandem with generative AI leveraging the best of both technologies, but you need the right software to combine the algorithms for true value.
With predictive AI, more specifically predictive modeling and predictive analytics, companies today generate real business value in the short to mid-term by predicting customer behaviors by enabling more effective and efficient customer engagement within the customer experience.
By using software like the Faraday predictive analytics and predictive modeling platform, you can have the AI infrastructure needed to predict customer behaviors like likelihood to convert, likelihood to churn, forecast spend, and product recommendations. With these predictions, you can drive data-driven customer experience use cases like AI lead scoring, churn prevention, adaptive discounting, and next-best offer.
Platforms like Faraday are real-world targeted AI, not some general-purpose AI platform for generating news headlines. Faraday focuses on predicting the behavior of individuals, with built-in Responsible AI, including features like bias mitigation and prediction explainability.
More real-world AI use cases
While improving the customer experience with predictive modeling and predictive analytics is a top use case for real-world AI implementation, there are several other primary use cases where AI is making significant inroads.
One of the areas already making a huge impact is predictive AI and generative AI being used to empower software developers, aka employee empowerment, allowing them to code quicker, improve time-to-market, and enhance their data with predictive algorithms.
Let's face it, existing developers are maxed out with work, and they can't build things as fast as companies want, plus they don't enjoy their coding jobs when they work on an assembly line. Today's AI coding assistants are intended to handle more routine tasks, allowing developers to focus on higher-value work. But to work well, they need to be combined with predictive AI engines like Faraday powered with Responsible AI.
"AI-augmented and machine learning (ML)-powered software engineering is changing the way software is being created, tested and operated, and the need for responsible AI is growing," said Dave Micko, Senior Director Analyst at Gartner. "Practices such as platform engineering will begin injecting insights from deployed systems into the systems being developed."
Conclusion
The AI hype machine has done its job. People are inundated with AI marketing to the point no one knows what is hype and what is reality. Yes, generative AI is exciting and has tons of future promise. But right now it's more in the demo stage of development.
While many generative AI apps do not need predictive algorithms, for many traditional business applications, using predictive AI plus generative AI can be a good optimization to move the needle on your business.
Predictive modeling and predictive analytics are where businesses need to put their technology investment dollars to start to see real returns from AI, in the short-to-mid term.
But how a company moves forward in this process is critical. AI must be done safely and responsibly to ensure that the consumer's, business's, and community-at-large best interests are maintained.
Ready for easy AI agents?
Skip the struggle and focus on your downstream application. We have built-in sample data so you can get started without sharing yours.