Noticed a change in your score reporting? Here’s how score aggregation is improving your results
Score aggregation improves prediction accuracy and stability by averaging scores from similar groups rather than estimating individual scores from aggregated traits.
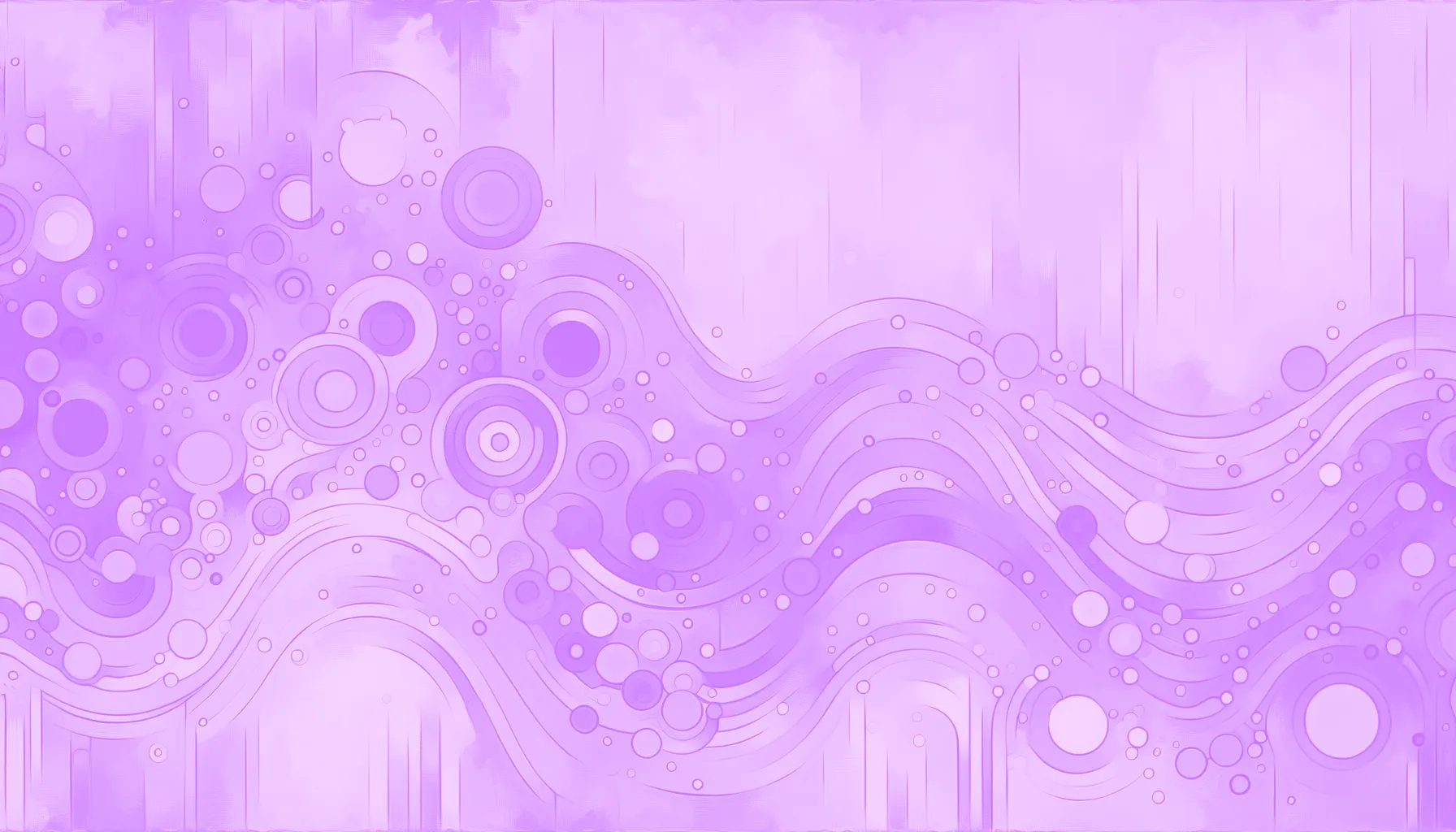
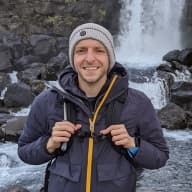
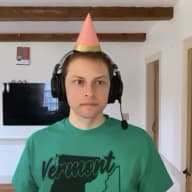
Score aggregation is a method we use to enhance the accuracy and stability of predictions when individual-level data is incomplete. Instead of relying solely on aggregated traits—an approach that works great when appending data but can introduce volatility in model outputs when scores are produced with these aggregated inputs—we take the next step and aggregate scores themselves. This ensures that models can still generate high-quality predictions, even when full individual identity resolution isn’t possible.
By leveraging aggregated scores, we can create smoother, more consistent predictions across varying levels of enrichment. This builds on our existing strategy of using aggregated traits for data appends but tailors it specifically for improving modeling outcomes.
A simple example
Sounds a little complicated? It’s actually easier than you think! Let’s break this down with a simple scenario that unpacks two hypothetical individuals:
John Doe – Fully identified
John lives at 456 Oak Street, and we have all the necessary PII to match him confidently in our system. Because of this, we can generate a highly individualized score based on his specific traits and history no problem. This is how our identity resolution process ideally works.
Jane Doe – Partially identified
Jane, on the other hand, lives at 123 Main Street, and we don’t have enough personal information to fully match her. However, we do know a lot about her household. Previously, we would have used aggregated traits from the household (or street, postal code, depending on how far out we’d need to go to find accurate information) to generate her score, but this could result in fluctuations.
Now, instead of scoring her based on those aggregated traits directly, we leverage an aggregated score—we average the scores of people in similar groups (in this case her household) rather than creating an average person based on traits and then scoring that, itself. This provides a more stable and accurate prediction, reducing potential variability and improving reliability.
So how does it affect you?
The key impact of score aggregation is that it makes every model better. However, you might also notice changes to individual scores, and here’s why:
- More reliable predictions: Instead of introducing potential spikes from scoring based on aggregated traits, we now rely on aggregated scores, which reflect a more stable signal.
- More inclusive modeling: Even when we can’t fully identify an individual, we can still provide a meaningful prediction using data from their household or surrounding population.
- Fewer gaps in data: If a person’s identity is incomplete, we don’t discard the opportunity to score them—instead, we use the best available aggregated score to fill in the blanks.
Key considerations
When implementing score aggregation, there are a few key considerations to keep in mind. First, using aggregated scores helps prevent sudden shifts in predictions that might occur when only aggregated traits are used. This approach provides a more stable and reliable signal. Second, our methods adapt based on the available data: when full PII (Personally Identifiable Information) is available, we prioritize direct individual scoring. However, when only partial data is present, aggregated scores serve as a reliable fallback. Lastly, if only household-level data is available, rather than estimating an individual’s score based on household traits, we derive a score from the averaged predictions of others in similar households, ensuring that the prediction remains accurate and meaningful despite incomplete individual information.
In conclusion
By adopting score aggregation, we ensure that models remain robust and useful even when some data points are incomplete. This helps businesses make better decisions with confidence, regardless of data availability. If you'd like to learn more about how this—and the numerous other services and AI agents that Faraday provides—could benefit your business, reach out!
Ready for easy AI agents?
Skip the struggle and focus on your downstream application. We have built-in sample data so you can get started without sharing yours.