Why you need predictive lead scoring today
Predictive lead scoring uses machine learning to analyze large datasets and prioritize leads most likely to convert, enhancing efficiency and effectiveness for marketing and sales teams. By leveraging AI, businesses can improve conversion rates, shorten sales cycles, and ensure alignment between marketing and sales, provided they maintain high-quality data and regularly refine their predictive models.
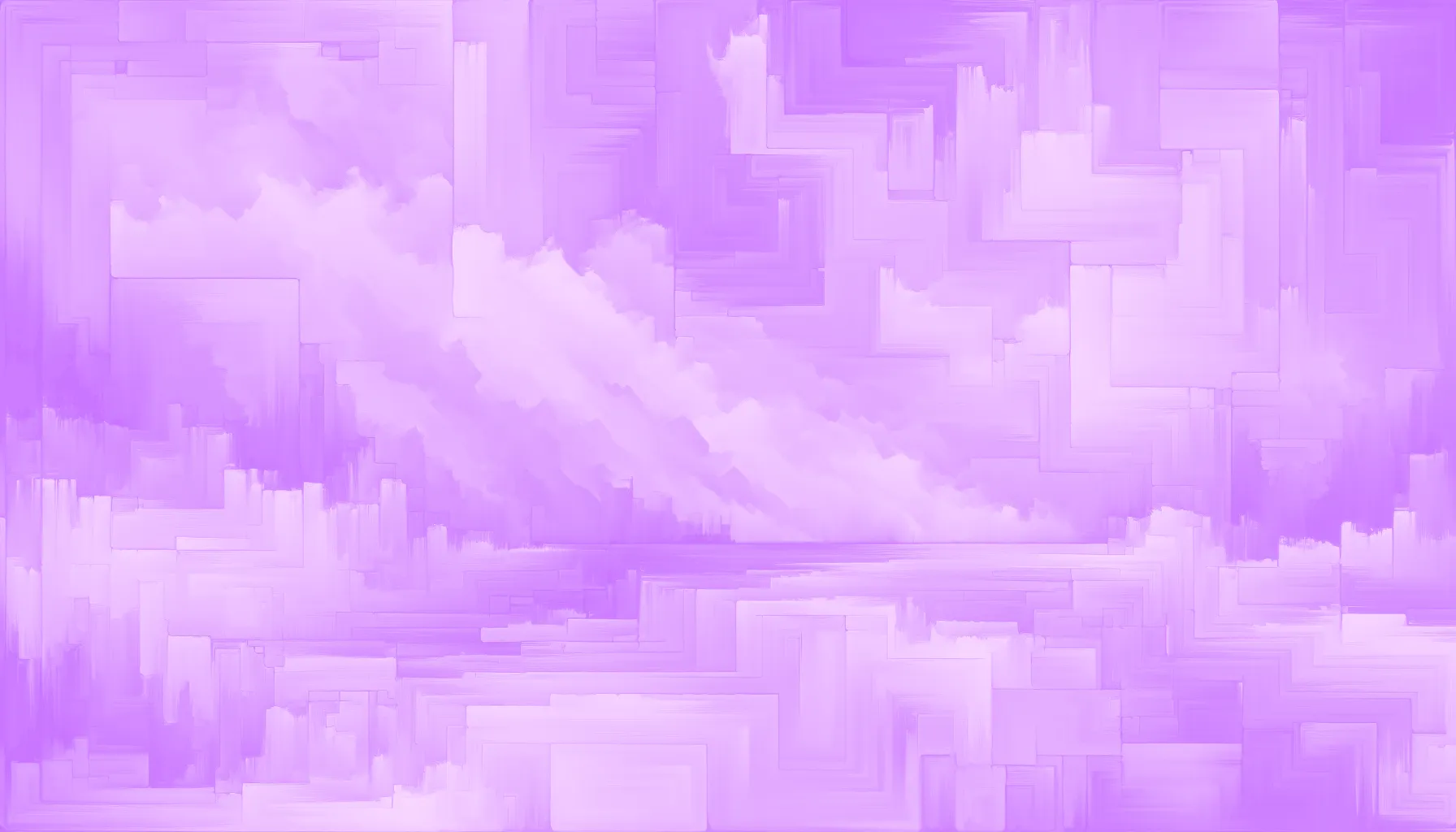
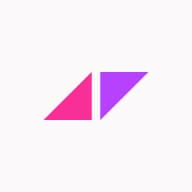
When you are marketing and selling consumer products, every lead counts. However, not all leads are created equal. As your business matures, it's crucial to move beyond simply filling your pipeline to effectively prioritizing leads that are most likely to convert. This is where the power of predictive lead scoring comes into play.
What is lead scoring?
Lead scoring is the method marketing and sales departments use to rank the quality of a lead, typically in the form of a numerical point system. Leads get scored based on attributes critical to the business, including demographics, transactional behavior, and customer engagement, giving sales professionals the most promising potential customers to contact. Lead scoring systems are fed with past leads and historical data to inform the current scoring model and are typically handled within an enterprise's CRM system.
But traditional, manual lead scoring has its drawbacks. It's time-consuming for employees who could be spending their time in more productive ways, and it can result in best guesses and other types of inconsistencies in the application of the methodology.
What is predictive lead scoring?
Predictive lead scoring represents a huge step forward for marketers and sales departments for qualifying leads at scale. By using machine learning algorithms, organizations can analyze enormous amounts of data and identify patterns to sort and prioritize leads, improving the quality of lead scoring mechanics. Predictive AI engines can ingest anything from CRM data, online data, IoT data, and behavioral data to help predict what leads will be the most likely to convert into customers.
During challenging economic times, budgets are tight, and sales and marketing teams are looking for ways to reduce costs and improve the efficiency and efficacy of their sales funnels. By leveraging AI-driven predictions, businesses can convert more leads by using those predictions to optimize, prioritize, route, and engage leads. Marketers can anticipate each lead's behavior in their funnel by infusing AI into their lead-handling workflows.
Predictive lead management works better than traditional lead management. The challenge is good predictions require robust and accurate data, which means resolving every detail down to the identity level. Only the right predictive software infrastructure will be able to address the data-oriented challenges to deliver effective predictive AI lead scoring.
The benefits of predictive lead-scoring
With predictive lead scoring, organizations can expect to see a variety of benefits. They will be more data-driven overall, leveraging not just observed data, but predictive data to improve algorithm performance. You will get better efficiency in your prospecting with better conversation rates and shorter sales cycles. Plus, you can look forward to an improved alignment and democratized data connection between marketing and sales establishing a single source of data-driven truth for both departments to rally around.
Three kinds of predictive lead scoring to consider
There are different types of predictive lead scoring, so it's important whatever software you choose can accomplish these three primary activities.
- Lead rejection — Does your organization purchase leads from lead generators and/or aggregators? Many of these leads are not worth buying. If your lead platform supports it, you can use predictive lead scoring to reject leads that don't meet a minimum conversion probability threshold, saving you significant time and money.
- Lead suppression — Does your organization spend money engaging every lead—for example, with direct mail—whether self-generated or purchased? Not every lead is worth the expense. You can use predictive lead scoring to suppress leads below a minimum conversion probability threshold—another way to save significant time and money.
- Lead prioritization — Does your organization engage your incoming lead funnel with a resource-limited sales team? In many cases, this means not every lead can get the same attention, and few can get the kind of attention and engagement you know will maximize conversion. You can use predictive lead scoring to ensure your best leads get handled first and with the most robust level of engagement. This is another way to boost revenue with predictive lead scoring.
The challenges of predictive lead scoring
One of the top challenges of deploying any type of predictive engine is making sure it is fed with quality data. The accuracy of the predictions will be driven directly by the quality of the data input into it. As the old saying goes, garbage in, garbage out (GIGO). You must have the right software platform necessary to make sure that data is complete, consistent, and accurate.
The other primary issue with predictive AI lead scoring is there is an ongoing process that involves the consistent refinement and retraining of data sets so that the predictive models remain accurate as new data comes in. These patterns can change over time.
Faraday delivers predictive lead scoring
Patterns that predict behavior in the early stages of a customer journey eventually stop working and new ones take over. Only Faraday uses time-linked model ensembles to tell the true customer story. Faraday detects and selects the best tools for the job, from time-tested algorithms to cutting-edge generative AI Everything you need to predict customer behavior is built-in to the platform. With Faraday's rich, built-in data, you can skip expensive licensing deals and start predicting behavior immediately.
Faraday provides the data and the predictive AI modeling infrastructure (IaaS) that enables organizations to iterate on their business challenges, instead of their technology infrastructure. With our platform, brands, and the software companies who support them, can now develop end-to-end customer experiences, at scale, driven by predictive insights.
In the end, a data-driven CX is a better CX. Faraday is the only predictive AI platform, both for software teams and brands, dedicated to predicting customer behavior. Everything you need to make customer experiences predictive is built into our software.
Ready for easy AI?
Skip the ML struggle and focus on your downstream application. We have built-in sample data so you can get started without sharing yours.