Using third-party data in marketing responsibly
Using third-party data responsibly benefits brands and consumers alike. Learn how brand marketers are doing it right.
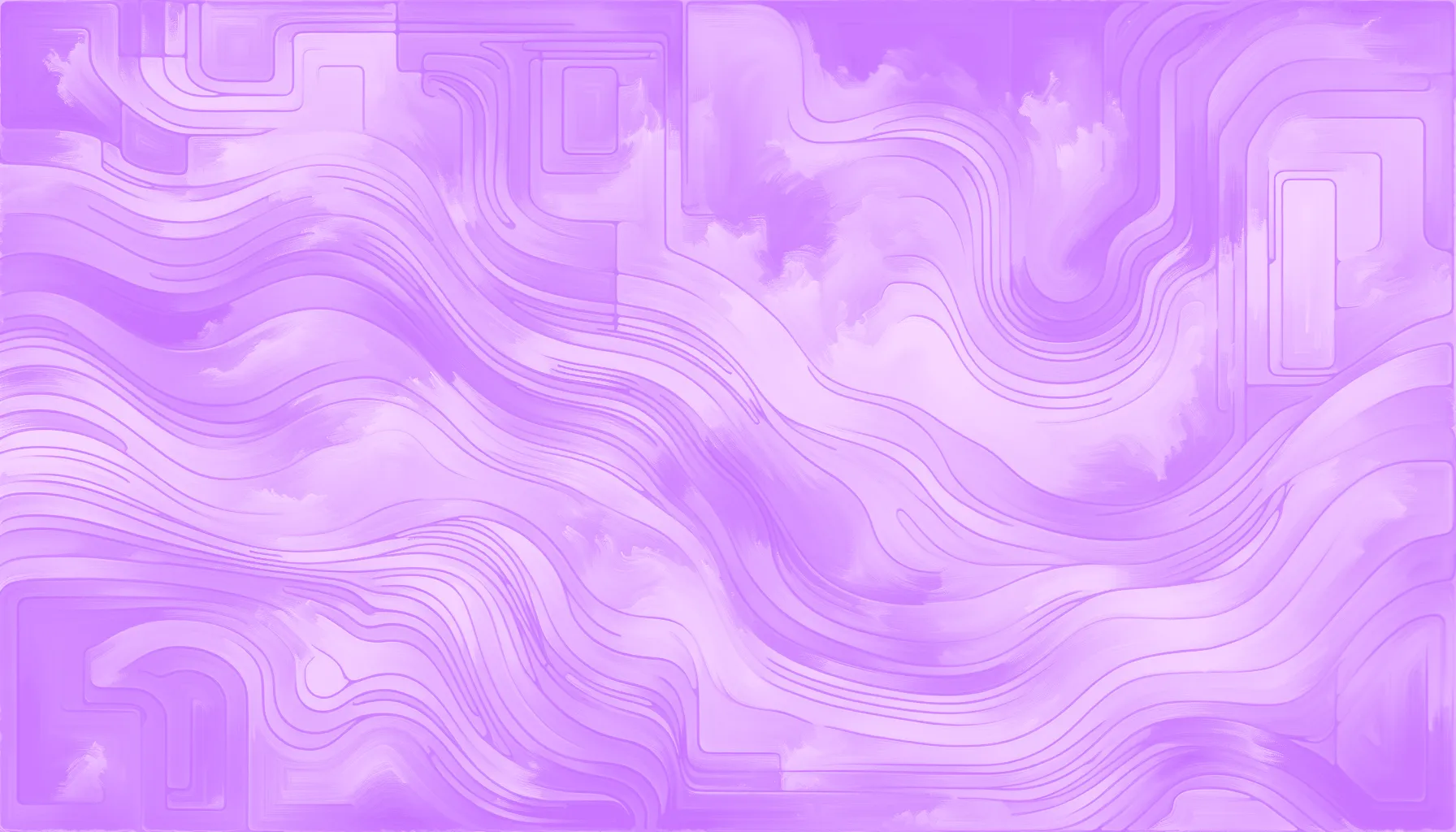
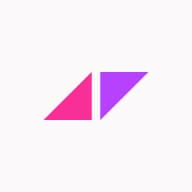
The use of third-party data isn't new, but it's becoming a necessity as businesses shift to a customer-centric approach. Marketers everywhere are realizing that first-party data is only the tip of the iceberg when it comes to understanding their customers.
The rise in digital technologies and interactions has increased the need for bringing outside data into their companies. While it may be as simple as understanding your existing customers' household and financial standing, with third-party data you can also gain deep insights into your leads on a known-identity basis.
Overall, leveraging third-party and first-party data adds breadth and depth to your customer insights, informing better branding and messaging decisions, personalization efforts across channels, and taking much of the guesswork out of targeted and location-based marketing campaigns.
Customer identity resolution
The capability to bring together first-party data from various sources and third-party data to compile a unified picture of each customer is increasingly valuable. Spending dedicated to identity data assets grew by more than 50% in 2018, to a total of $846MM.
Personally identifiable information (PII) is crucial to connecting the dots across multiple touchpoints (online and offline) to form a holistic picture of your customers and prospects so you can reach them with content that will resonate with them through the channels that matter.
Using third-party data for personalization
In a time when almost all consumers prefer ads and products that are relevant to them, knowing how and when to serve your target market is crucial to effectively engaging your customers. According to Forbes 46% of marketing executives are not where they want to be in terms of delivering personalization, and 57% say that they hope to advance their personalization initiatives over the next year.
Enriching your first-party data with third-party information on your customers' financial, household, transactional, or lifestyle categories gives you a clearer idea of what your customers look like in real life.
To take it a step further, enriched customer data helps machine learning algorithms build better models. Cluster models, for example, sort individuals into distinct groups based on common attributes. These groups, or personas, can affirm your intuitions about what your customer base looks like, but may also surprise you by revealing significant groups you hadn't known about or previously considered important.
Using third-party data for ad optimization
If you're using predictive lead scoring capabilities, the accuracy and precision of those scores improve with the quality and quantity of third-party data that you introduce. This not only benefits your leads' experiences, as they'll receive content that better aligns with their interests and where they are in their customer journeys, but it will save you time and money when you're deciding how to prioritize your outreach.
A popular way of leveraging third-party data with predictive modeling for digital marketing is Facebook lookalike audiences. But since the platform shut down advertisers' access to this data in April of 2018, gaining deep insights from Facebook has been a struggle for many businesses that are trying to better understand their audiences and target the right consumers.
If you enrich your first-party data with third-party attributes, you can gain insights on a granular level that you aren't getting with Facebook and other marketing channels. You can then segment known-identity audiences based on certain attributes — or develop in-depth personas for segmentation — and push the appropriate ad creative to those individual segments with the goal of increased, personalized engagement. This goes for not only new leads you're nurturing, but also for the customers you're actively engaging.
Another advantage of leveraging third-party data is the ability to match site's captured emails to real people. This allows you to bucket leads into certain personas for more personalized nurture campaigns that will pull them through their customer journeys with you. Your leads are more likely to receive messaging that aligns with where they are in their individual journeys, and it will save your team time and money when you decide how to prioritize your lead outreach.
Using third-party data for location-based marketing efforts
Geographic data has obvious benefits, but incorporating additional third-party in geographic analyses improves the quality of the insights. Knowing where your ideal customers live is one piece of the geomarketing puzzle, but gaining an understanding of how your audiences will best interact with your ads offline is achieved by knowing more than just their geographic location.
Attributes like household information, transportation preferences, and lifestyle data helps you prioritize where you place ads, as well as where you might establish new brick-and-mortar presences. A subway ad or billboard placed in your customers' neighborhoods can be a great way to advertise your e-commerce business for those who don't live near a storefront, but for customers who are in your brick-and-mortar trade zones, perhaps a direct mailer advertising an in-store sale would better drive business.
From a creative perspective, audiences can vary widely depending on their physical locations, so the messaging and images chosen for your campaigns should be curated for specific audiences in particular geographies. Providing relevant content increases your chances of audience engagement, so capitalizing on the insights provided by third-party data is a necessity.
This is the case with SEM advertising, which segments audiences by geographic location. With the insights gained from third-party data, you can optimize your SEM spend by targeting areas where higher-value customers reside, spending less on areas that aren't as likely to bring in revenue.
Using third-party data responsibly
With the rise of regulations like GDPR, privacy and validity of data are prominent concerns when using third-party data and PII. However, there are ways to ensure that your customers' and prospects' privacy is protected. You want to be sure your data comes from a verified source, isn't dangerously invasive, and is leveraged to benefit the customers you're targeting.
Social scraping is a common tactic, and it can provide large categories of data on behavioral tendencies, consumer habits, and some personal information, though this data may not always be entirely accurate. At Faraday we avoid social scraping in favor of canonical sources of information from established third-party data vendors. Vendors like Epsilon verify the accuracy of the high-quality data we license and leverage for our partners.
Faraday's Director of Data Science, William Morris, notes that we sidestep many consumer privacy issues by dealing with datasets that categorize data, such as consumer purchases, by type of product and frequency, rather than the exact stores where those purchases are made. This leads to a significant lift in machine learning models' predictive capabilities without being invasive from a privacy standpoint. Additionally, each of our clients' customer data is siloed using industry-standard practices to protect the privacy of each clients' customers.
Faraday also actively works to build models that are agnostic to a person's membership to protected classes. We exclude potentially harmful categories like ethnicity, religion, or primary language data, as these can disenfranchise certain groups of the consumer population. As an additional security measure, we employ a suite of tools for post-model checking to ensure that attributes that could indicate a person's race or class don't make the models biased or adversely impact a population.
Taking into consideration these types of ethical practices is important when exploring your own use of third-party data.
Ready for easy AI agents?
Skip the struggle and focus on your downstream application. We have built-in sample data so you can get started without sharing yours.