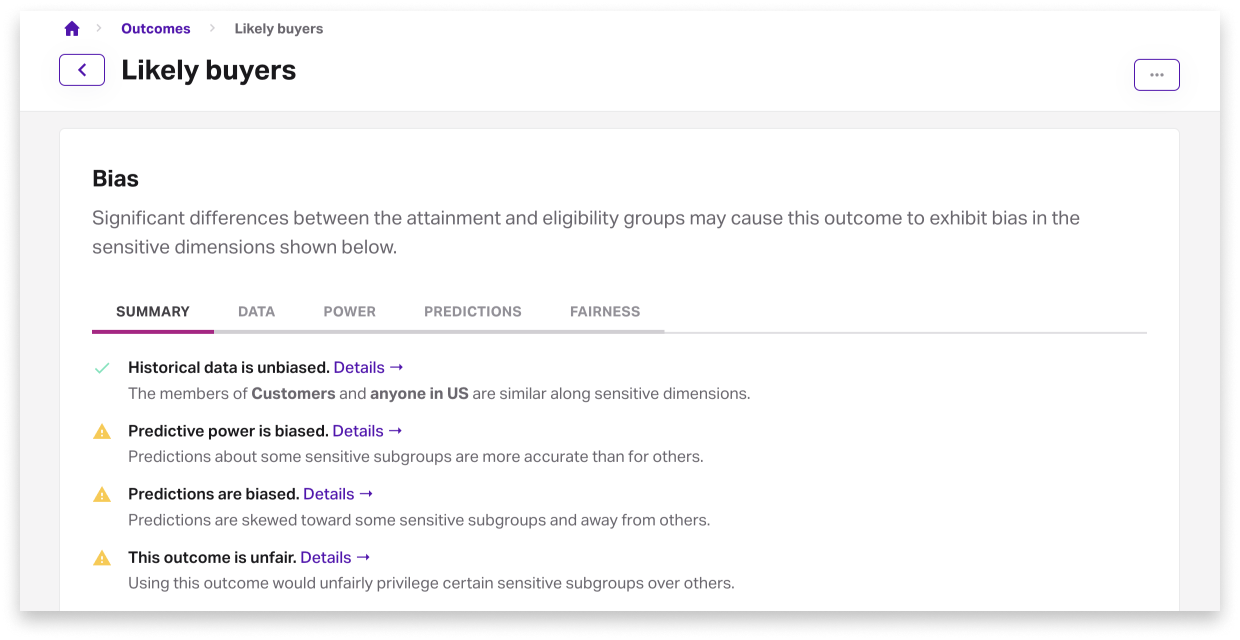
Introducing Faraday bias management
3 min read
One of Faraday’s founding pillars is the use of Responsible AI, which includes both preventing direct harmful bias and reporting on possible indirect bias. We’ve been building these features directly into the platform over the past several months, and today we’re going to walk through two new features in Faraday with bias reporting and bias mitigation.
Bias reporting
To see the bias report on an outcome, head to outcomes and scroll down to the bias section to see the breakdown of bias discovered in the data, power, predictions, and fairness categories, which we'll dive into below. These can also be retrieved via the API using get outcome analysis.
Faraday uses four categories to report on bias for an outcome:
- Data: Measures selection bias in the underlying cohorts used in the outcome. In a Faraday outcome, two labels exist for the purpose of this blog post: positive, or the people from the attainment cohort that were previously also in the eligibility cohort, and candidate, or the people from the eligibility cohort.
- Power: Measures outcome performance on a subpopulation compared to baseline performance–for example, Faraday will compare how well the outcome performs on the subpopulation “Senior, Male” compared to everyone else.
- Predictions: Measures proportions of subpopulations being targeted compared to baseline proportions in order to see if the subpopulation is over or under-represented.
- Fairness: Measures overall fairness using a variety of common fairness metrics in the literature. For example, Faraday will look at whether or not the subpopulation "Senior Male" is privileged or underprivileged.
Each bias category uses groups of people called subpopulations, which are combinations of people defined in the understanding bias reporting section's sensitive dimensions dropdown table. Examples of these are "Teens with gender unknown" and "Senior Females." We're launching with two of these sensitive dimensions in age and gender, but will be rolling out more over time.
If you discover that one of your outcomes includes harmful bias, you can create a new outcome using the same cohorts, but enable bias mitigation options while creating it to compare results.
Bias mitigation
When creating a new outcome, you can choose to mitigate bias by using the toggles in the bias section. Check out our outcomes documentation for info on different bias mitigation strategies.
🚧️Taking action on bias
Mitigating bias isn't something you should set-and-forget, meaning it's not recommended that you toggle both age and gender on for every single outcome you create. For example, if you're a womens' swimwear brand, your data will skew heavily toward women, and mitigating gender would negatively impact your lift.
Always keep the populations that you're making predictions on in mind when considering bias. In the above example, it's totally fine to ignore the biased skew towards a certain gender or age group because they're your ideal customers.
We're thrilled to continue to drive Responsible AI forward by providing agency for our users to both discover and mitigate bias in their customer predictions. Stay tuned for a deep dive into the science behind how Faraday determines bias.