Removing the black box around customer predictions with Faraday score explainability
Learn how every individual you make customer predictions on had their score calculated.
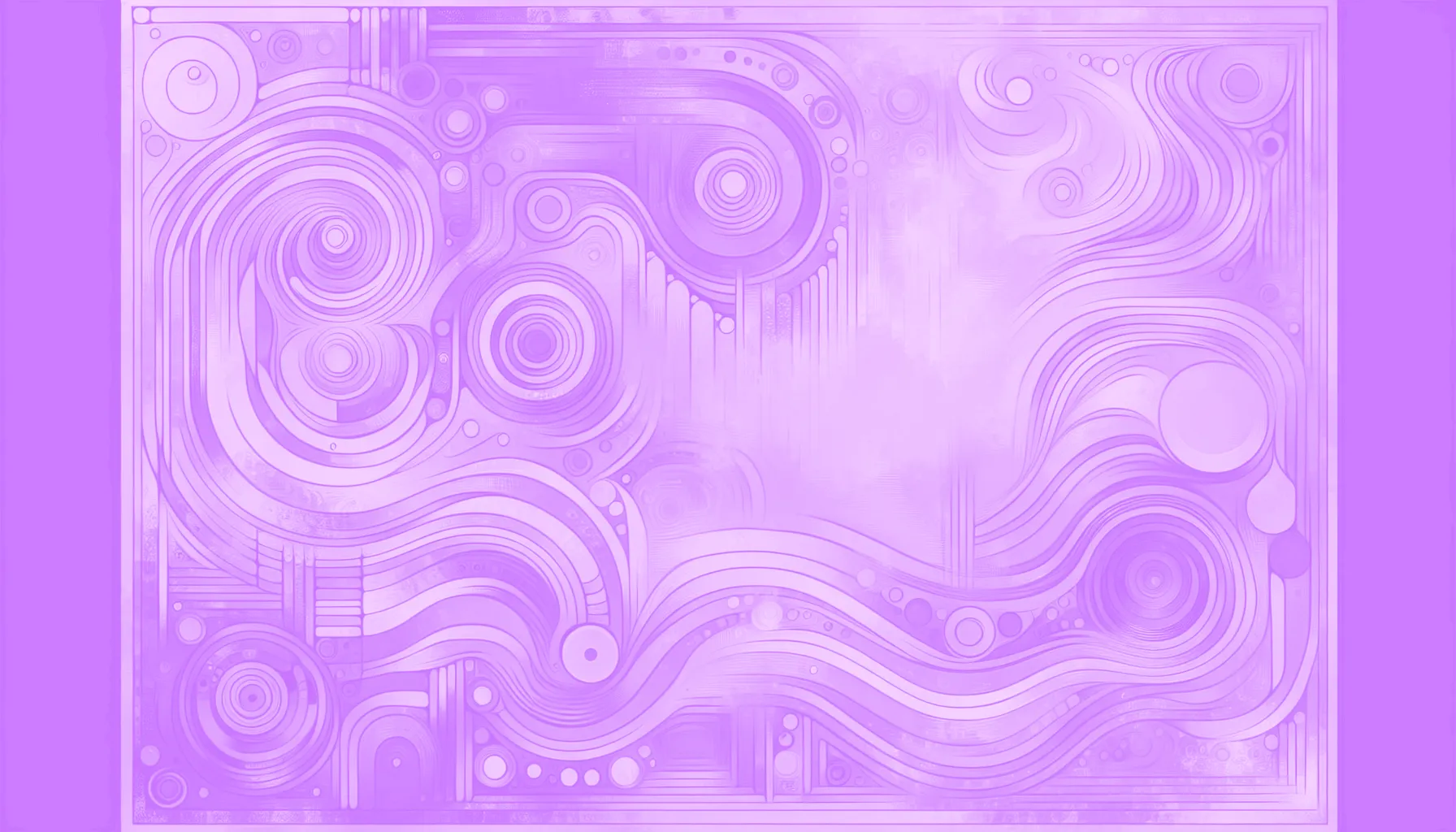
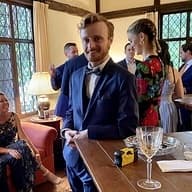
AI is on everyone’s minds due to its myriad use cases and applications across industries. From chatbots getting people where they want to be faster, to providing financial planning advice, to using ChatGPT to help out with a resume or piece of code, to making customer predictions for likelihood to take an action and personalization–AI isn’t going anywhere anytime soon.
64% of businesses feel that various aspects of their organization will see increased productivity due to AI, and it’s no surprise when you think about the countless tasks that people have been doing for ages–segmentation, data analysis, and so on–and how much time & effort is saved by offloading them to AI. The use of AI for generating content in particular has scaled so fast that discussions abound on the impact that this AI revolution can and will have on human jobs, and brands like NPR and MIT are publishing guides for how to spot AI-generated content.
The black box problem
The issue at the heart of this AI quandary is known as the black box problem. In short, the problem is that while what we’re seeing AI accomplish is incredible–and seemingly accurate in many ways, at first glance–by and large, we don’t understand how it reaches its particular conclusions, and what data it uses to reach them.
Faraday has long pursued making AI that uses responsible data to make responsible predictions accessible for all, and part of this pursuit has been tackling the black box problem. Starting today, every customer propensity prediction made using Faraday infrastructure can include explainability metadata.
Explainable AI now in Faraday
Sounds good, but what does this mean?
Faraday has always provided detailed technical reports that analyze the impact of key data points on predictions at the overall level.
Now, each prediction, down to the individual level, has its score explained by the features in your data that had the highest impact on that individual’s prediction.
In the above example outcome, we see that, overall, the approximate number of children and age are the most predictive factors. And we can see what ranges lead to what average impact. For example, people with no kids have—all else equal—a slightly higher probability than those with 1–2 kids. This kind of aggregate reporting has always been available in Faraday.
Above, we see an example of CSV output from a pipeline. John is impacted by this outcome’s major factors, age and number of children, and leaves him with a low probability.
Jane, on the other hand, exhibits a more unusual combination of traits that give her a much higher probability for different reasons. For Jane, both her household income and millennial lifestyle saw her conversion probability higher than John because this business often sees conversions from people with those traits–even if they’re not the dominant traits.
Explainable AI is Responsible AI
The central idea of Responsible AI is to understand our models—how they work, why they produce the results they do—enough to identify and correct their shortcomings. That’s why we have invested so heavily in, for example, bias detection and mitigation here at Faraday.
But even as we develop a strong understanding of and confidence in our models in the aggregate, we must not lose sight of the impact that prediction can have on individuals. We should demand nothing less from our AI models than that they explain themselves. Explainability is as core to Responsible AI as transparency and fairness.
A simple statement that explains a given prediction may not seem like much at first glance, but we feel otherwise. The just trust us hand-wave that has been the motto engraved on the black box around AI for decades has been preventing our industry from moving forward with respect and responsibility for businesses and people alike–until now. Now you can see exactly what data helped AI reach the conclusion that it did, and feel confident that you’re making responsible predictions with responsible data.
Ready for easy AI?
Skip the ML struggle and focus on your downstream application. We have built-in sample data so you can get started without sharing yours.