How to optimize ad targeting and comply with financial advertising regulations
In finance, remaining compliant to fair lending regulations doesn’t mean you need to accept worse acquisition performance. Learn how to use AI and third-party data to optimize ad spend by surfacing your most likely-to-buy customers in under 15 minutes, while ensuring you're compliant to any relevant regulations.
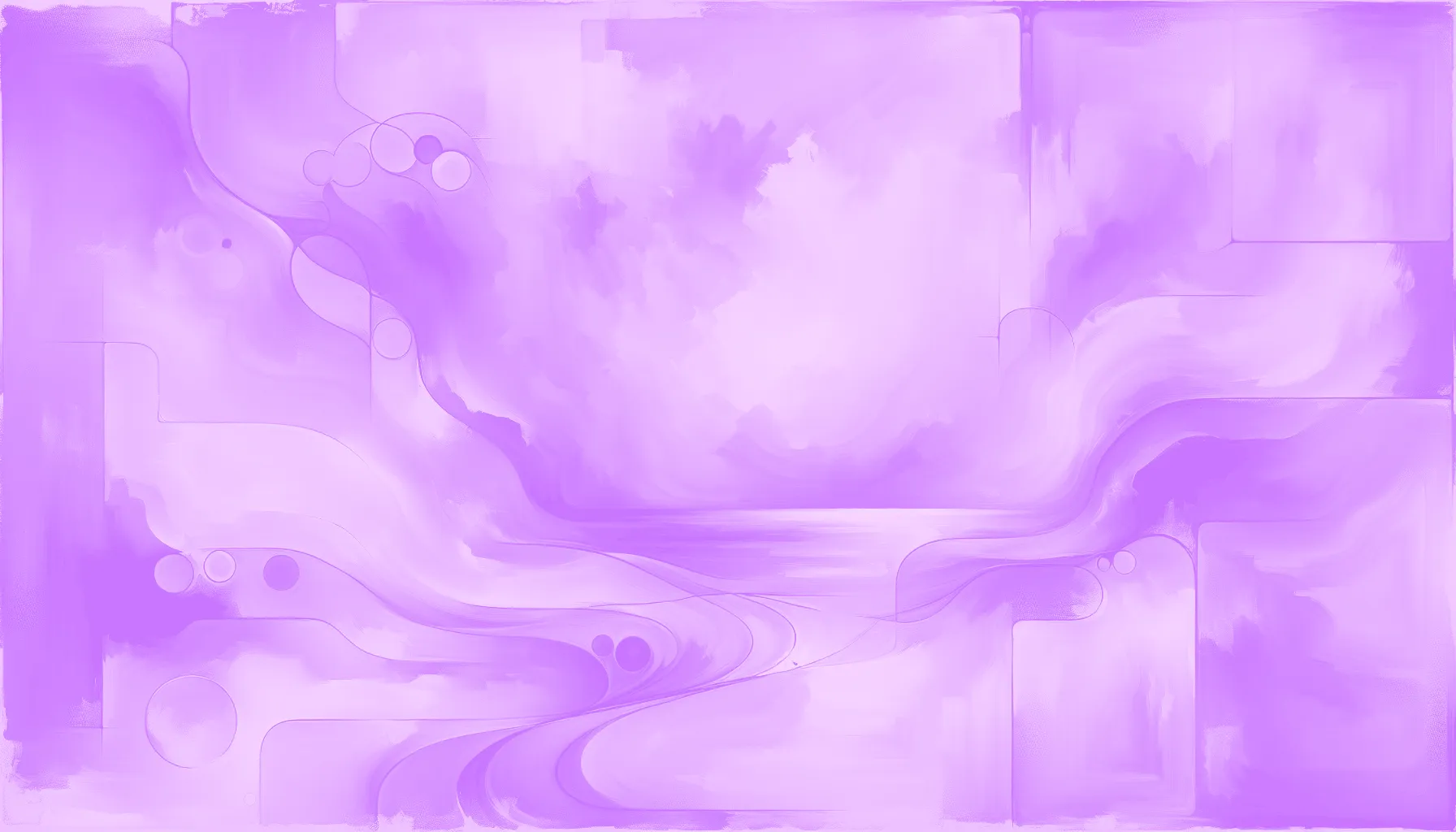
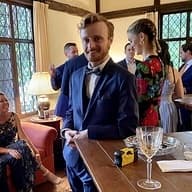
If you’re a marketer in the finance industry, you know you have some heavy regulations to work with on Google, Facebook, and other platforms, where consumer protections are put in place to help prevent discrimination. These regulations can make it difficult to reach your ideal customers when targeting new audiences, which likely means that you’re spending more than you need to on inefficient acquisition.
Complying with financial advertising regulations doesn’t mean you need to throw in the towel and accept worse performance. You can respect your customers’ privacy and be smart with your marketing strategy at the same time–you just need to think out of the box. That’s where built-in third-party consumer data and responsible AI come in.
The data you have on your customers might be good, but purchases, adoptions, and clickstreams aren’t robust enough to give you a true leg up on your competitors, nor is it enough to ensure you’re not unknowingly discriminating against protected classes. Third-party data, when unified with your first-party customer data, can give you a 360º view of your customers, leads, and virtually any other group of people your business cares about.
With this breadth and depth of data in your pocket, you can then use AI to optimize your ad spend by surfacing your most likely-to-buy customers while remaining compliant by:
- Excluding protected classes from your predictions to ensure no unintended bias
- Targeting at the right aggregation level for your industry (counties, rather than zip codes, for example)
- Removing both human bias and error from the equation by using AI
- Monitoring and minimizing bias in predictive models through bias reporting
Faraday enables you to do all of these things–without a data science degree or programming knowledge–and in under 15 minutes. Here’s how.
Connect your customer data to combine it with third-party attributes
To start, you plug in last quarter’s adoption data in by mapping the data in Datasets.
Next, you use this new data to define a cohort–a group of people you’re interested in–of these new adopters.
Build a predictive outcome to identify likely buyers
Last, you follow the recipes for likely buyer predictions in Google Ads and Facebook to create your likely buyers outcome, selecting your customers as your attainment cohort because that’s your end-goal–you want people to become customers and enter your customers cohort.
Exclude protected classes with trait blocking
When describing your outcome, you choose trait blocking to remove protected class traits. This ensures you’re not only complying with financial fair lending regulations, but are also using AI responsibly.
Choose the right aggregation level for your likely buyer predictions
Once your predictions are ready, set your aggregation level based on how high you’d like to zoom out of a location–such as by county to remain compliant with Google’s restrictions on zip code targeting.
Push high-scoring locations to your ad platform
With your highest-scoring locations in-hand, you plug them into your Google Ads campaign:
And your Facebook campaign:
That’s it! Your best-bet locations are plugged into Google and Facebook, and fewer than 15 minutes were spent making your predictions.
Real results from a customer in the financial services space
A Faraday client in finance recently followed these exact steps to kick off a new acquisition campaign for an underperforming insurance plan, and they’re expecting a 3.2x lift in conversion rate in the top 10% of individuals alone.
Next up for them is adding direct mail into the mix, but the possibilities are endless.
Sign up free today.
Ready for easy AI agents?
Skip the struggle and focus on your downstream application. We have built-in sample data so you can get started without sharing yours.