How to target direct mail campaigns using Faraday
How to use each feature in Faraday to create predictions that enable you to accurately target direct mail campaigns through propensity predictions.
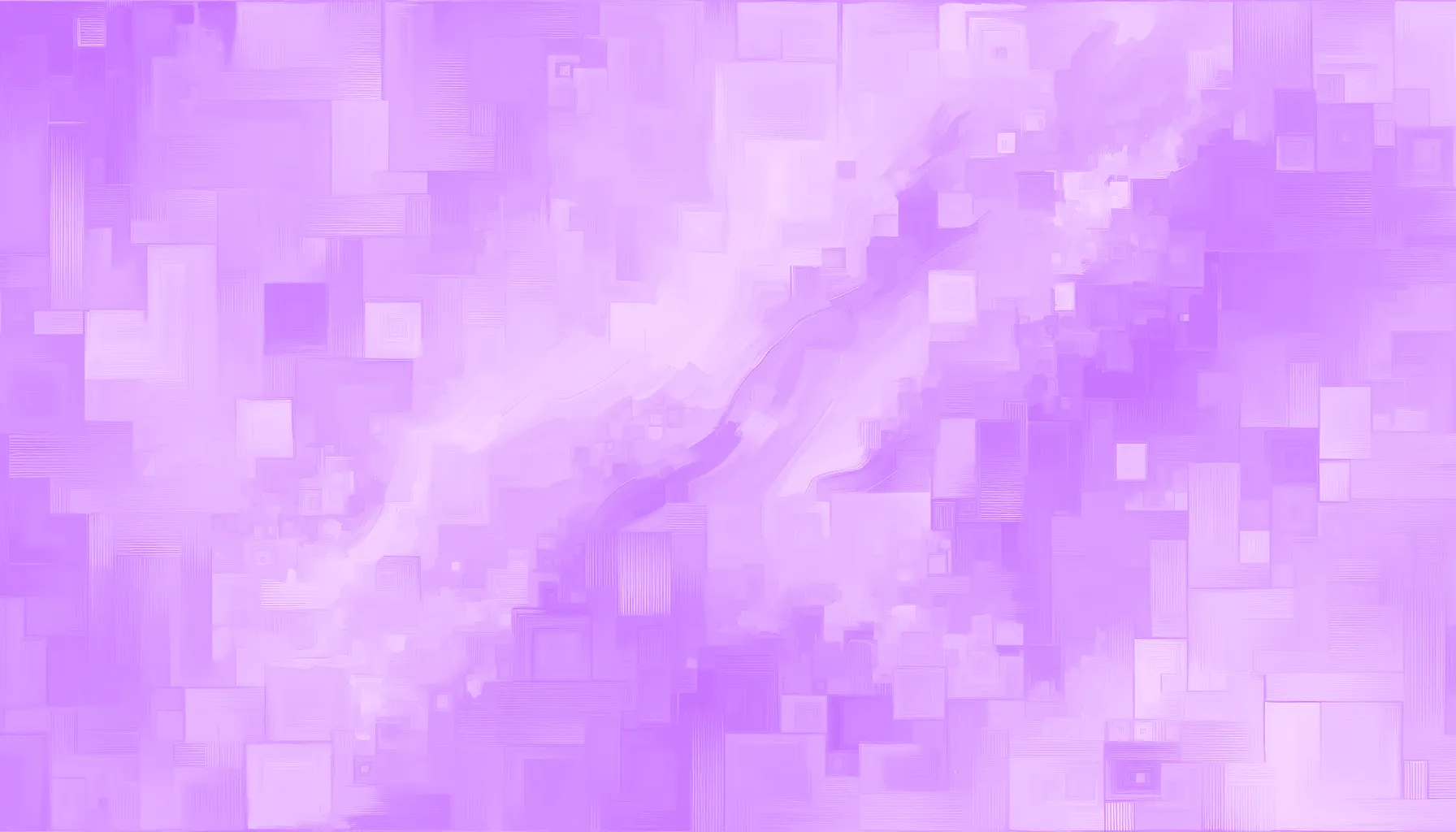
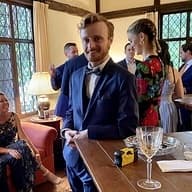
It’s no secret that personalized direct mail marketing is a great way to engage with your customers. Whether you’re interested in driving repeat purchases from current customers, finding new ones, winning back old ones, or something else entirely, direct mail has proven to be an increasingly effective strategy to make your brand stand out in an era awash with the same old email marketing tactics. In this feature guide, we’ll walk through how to use Faraday to enable direct mail marketing campaigns that are highly personalized, and highly effective.
How can Faraday be used for direct mail?
Faraday can be used for your direct mail campaigns by creating targeted cohorts, or groups of people, based on attributes from the Faraday Identity Graph, such as their ability to receive mail via, as well as their physical address. In this guide, we'll walk through how to create a cohort for direct mail, and how to use it when deploying a pipeline.
Creating a cohort for mailable U.S. customers
To create a new cohort:
-
Navigate to cohorts via the menu bar on the left-hand side and click + New cohort in the upper right.
-
Optionally, select an event for the cohort. In this specific direct mail use case, we're going to skip this step. For more info on this, check out our cohorts documentation.
-
Next, click the Add trait button, which is the most important part in designating that this cohort of people are able to receive your direct mail.
- In the Add trait popup, we're going to find & select the following trait:
- Record quality code: To ensure that the residence can receive mail at all. Choose the option to "select exact values from list" to select mailable.
Optionally, add a geography trait such as Postal code/ZIP to target specific locations. When using a cohort with a geographic restriction, like "Direct mailers in Burlington, VT," in a pipeline, the deployment will only include people in that locations.
- Enter a unique name for the cohort, like Direct mailers, and click Create cohort.
- Once finished, click Save changes. The cohort will begin building and will display with a calculating message.
Creating a pipeline to predict likely buyers for direct mail
While our Direct mailers cohort is building (if we select it in a pipeline, the pipeline will build once the cohort is ready), we're ready to create a pipeline and deploy it via CSV, which will let us plug our direct mail cohort right into our preferred mailer platform. For a more detailed look at creating a pipeline, check out the pipelines documentation.
-
Navigate to Pipelines, and click + New Pipeline in the top right to create a new pipeline.
-
In population to include, we'll grab the cohort we just created, Direct mailers, as we're only interested in the group of people we've selected: people in the U.S. who are able to receive direct mail.
-
In population to exclude, we can optionally add our Customers cohort if we’re only looking for net-new customers.
-
For our payload, we'll select:
-
Our propensity (likely to buy) outcome. This should be a standard likely buyers outcome, with your Customers cohort as the attainment, and Anyone in Faraday’s identity graph as the eligibility. For more info on creating that outcome, see the outcomes documentation. We’ll filter this outcome a little further on to target the top 20% most likely to buy people in this payload so that we're saving resources by not sending our direct mailer to people who aren't likely to buy.
-
Optionally, we can apply our customer personas to the people in this pipeline so that we can tailor creative & copy to their wants & needs.
-
- Enter a unique name, and click Save pipeline. The pipeline will begin building, as indicated by a loading bar. You'll receive an email when the pipeline has finished building.
-
Once the pipeline is complete, head to the Deployment section inside the pipeline, and select + Add under Hosted CSV.
-
We're presented with the choice of Hashed, Referenced, Identified, and Aggregated as options for our predictions. Since we're creating a direct mail pipeline, select Identified so that identities are not hashed, and we can see physical address information, and so we can filter by residence below.
- In Individual identification options, we'll select One row per residence (address) so that we don’t send mail to more than one person in a household.
-
Select human friendly for column headers that are easily readable, and click next.
-
Now we’ll expand filter, then click add payload filter so that we can specify that we’re only interested in the top 20% of likely buyers, ensuring we’re not wasting time and money on bad fits.
- In the payload filter, select propensity to buy - outcome percentile to sort by score percentile, or the distributions of propensity scores within the Direct mailers cohort we targeted.
- Change the operator to greater than or equal to (>=) and type 81 into the next field. Since the scoring is inclusive, this means that, in this deployment, we’ll filter it in a way that we only receive the scores for the top 20% of likely buyers.
If you have a specific budget to stay under, expand the limit option to add a cap on the number of rows you receive. For example, maybe each direct mailer costs $10, and you have a budget of $50,000. You’d set the limit to the top 5,000 rows.
- Last, click finish to complete the deployment, after which it will begin building. We can use the download button to download the CSV of a completed deployment for upload into our direct mail platform, which will include the top 20% of likely buyers that are able to receive mail in the country, (optionally) with their predictive personas included so that we can tailor copy & creative appropriately.
Ready to try it out? Create a free account.
Ready for easy AI agents?
Skip the struggle and focus on your downstream application. We have built-in sample data so you can get started without sharing yours.