Identity matching makes or breaks your predictive strategy
Your predictive model is only as strong as your data’s match rate—because if you can’t resolve who’s in your list, you can’t predict what they’ll do.
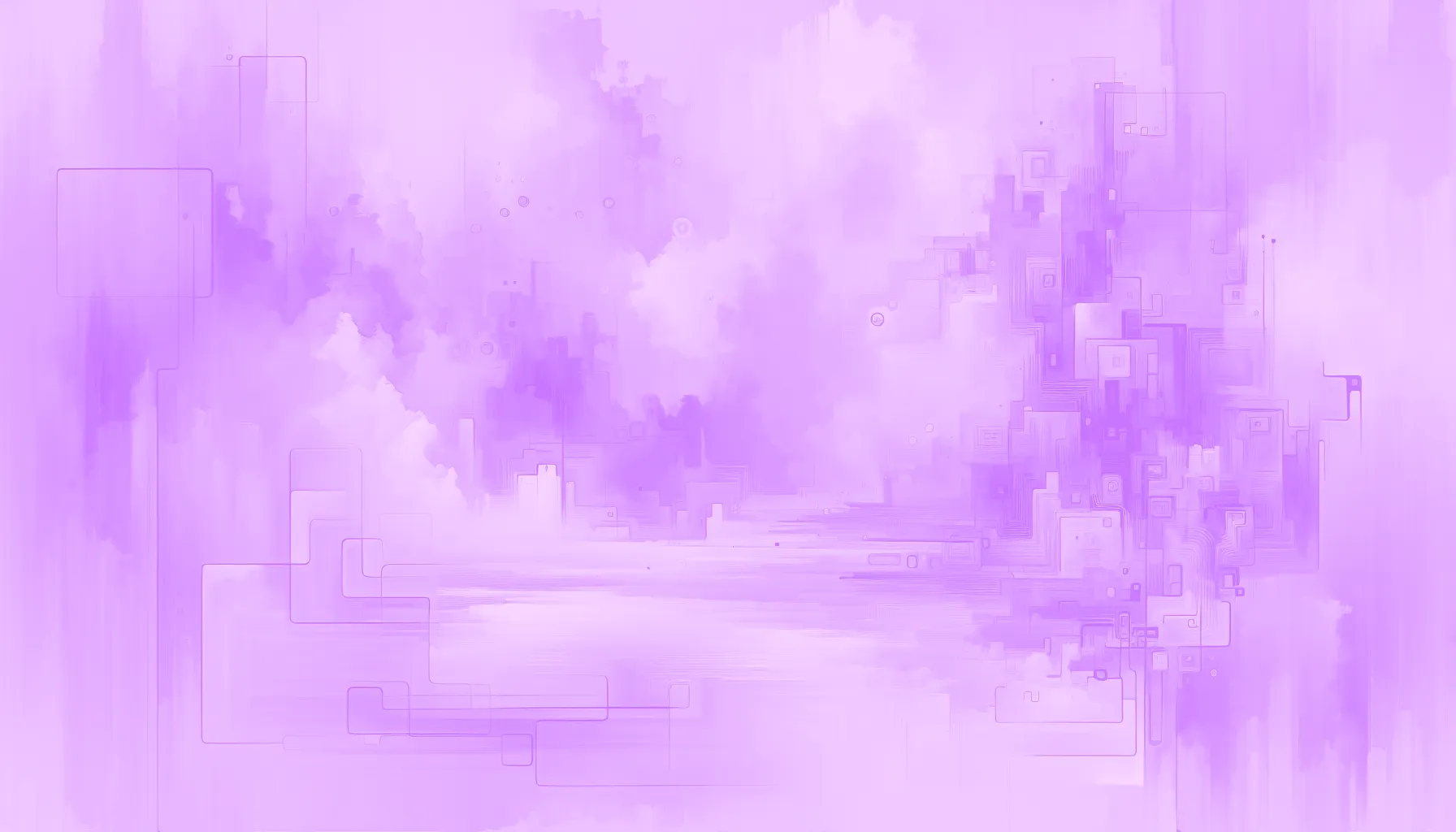
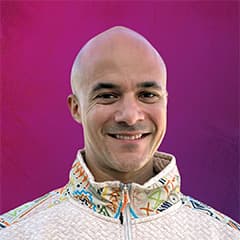
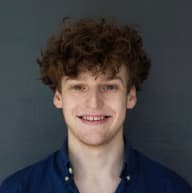
This post is part of a series called Getting started with Faraday that helps to familiarize Faraday users with the platform
You’ve got data—most likely lots of it. And if you’re the type of person who ends up on a Faraday blog, you’ve probably already considered the value predictive modeling could add to your brand. But not all predictions are created equally.
When does your data require enrichment?
For some tasks, modeling on first-party data alone—like transactions or web events tied to known users—is enough. This works well for optimizing things like cart abandonment campaigns, existing customer retention, or upsells within your CRM. If you determine that’s all you need at your company, Faraday can absolutely model on just your first-party data.
However, first-party-only modeling comes with a few major limitations:
- It can’t help you reach new customers who haven’t engaged with you yet
- It struggles with cold start problems when launching new products or campaigns
- It’s limited by the scale and diversity of your existing audience
- It can miss broader behavioral patterns visible only in third-party data
If your goals include reaching new audiences or launching into unknown territory, you’ll quickly find the cracks in a first-party-only strategy. That’s where Faraday’s data advantage comes into play.
We don’t just model on your existing data—we help you expand it. Through identity resolution, we enrich your records with insights from the Faraday Identity Graph (FIG), a privacy-safe dataset covering over 240 million U.S. adults. This allows us to bridge the gap between what you already know and the wider world of high-propensity customers you haven’t met yet.
Enrichment lets us go beyond who a person is, and start modeling what they’re likely to do.
But attentive readers will have noticed a word that might stand out: “enriched.” No, we’re not talking about flour, uranium, or even beer. In this context, enrichment refers to our ability to match the identities in your dataset to real individuals or households in FIG—so we can enhance your records with high-value predictive traits.
So what is identity matching, anyway?
Identity matching is the process of taking the first party records you give us—names, emails, phone numbers, addresses—and matching them to real people and places in FIG. It’s how we go from “some person” to “this person, at this residence, with these behavioral signals.”
Faraday supports two levels of matches:
- Person-level match: We recognize the individual based on identifiers like name + address, email, or phone, and enrich with person-level traits (e.g. age, gender, purchase propensity) and property-level traits (e.g. home value, square footage).
- Residence-level match: We recognize the household address, but not the individual. In this case, we can still provide property-level traits and aggregate averages for that address.
How the Faraday Identity Graph fits in
As noted, at the core of this process is FIG—our proprietary dataset, which we’ve been building over the last 12 years. This set covers thousands of behavioral, demographic, and psychographic attributes on over 240 million U.S. adults and their households. When we get a match, FIG is what powers the predictive features we feed into your model.
The inclusion of this set—and third-party modeling more broadly—solves some critical problems that first-party data alone can’t address:
- Cold start: FIG helps predict outcomes even when you have little to no historical data on a segment
- Audience expansion: You’re not limited to your CRM—you can model and target high-propensity lookalikes across the U.S.
- Signal density: FIG adds new, behaviorally-relevant features that enrich your model’s inputs
- Long-term accuracy: More diverse and representative data leads to more generalizable predictions
No match? No enrichment. And that means fewer signals to work with.
Why match rate matters
Match rate—the percentage of records we’re able to enrich—is a direct lever on how well Faraday can perform. It governs:
- The accuracy of your predictive model
- The reach of your campaigns
- The value of our insights to your team
Here’s what typical match rates look like, depending on the identifiers in your dataset:
Input type | Typical match rate |
---|---|
Name + address | 60–80% |
Email only | 30–50% |
Phone only | 5–10% |
If your list is heavy on phones or anonymized emails, that’s likely dragging things down. And if you’re seeing <50% match overall, it’s time to investigate.
Troubleshooting a low match rate?
One of the most common issues we encounter, and accordingly one of the biggest impediments we face when it comes to a good model is a low match rate. But sometimes diagnosing an issue isn’t as easy as you might expect. So here are a few common culprits to look for:
- Sparse or malformed identity data
- Hard-to-match populations (e.g. younger adults, recent immigrants, underbanked)
- Anonymized or junk emails (e.g.
email@email.com
, Apple relays, Doordash+ aliases) - Business names and commercial address (rather than personal records)
- Missing names, flipped fields, or swapped first/last names
- PO Boxes and apartment units with formatting issues
Sometimes it’s just formatting. Sometimes it’s about who’s in your list. But if your list looks like this, you probably have a guess where to start.
First name | Last name | Phone number | Last outreach date | |
---|---|---|---|---|
Rose, Benjamin | ben.rose@… | Faraday.ai | 434 54🥕4 | Went great 👍 |
What we do when match rate is low
When match rates dip below expectations, we kick off a diagnostic process. We look at the identifiers you provided, check for issues like junk emails or missing fields, and investigate how much of the dataset contains the high-value combo of name and physical address.
Depending on what we find, we might:
- Fix dataset mapping or formatting issues
- Ask for additional identifiers (e.g. billing vs. shipping address)
- Run a Match Boost through upstream vendors to attach new emails, phones, or addresses to help improve match
Heads-up: Match Boost isn’t always included in contracts, so we’ll confirm that before we proceed.
And if we’re getting solid residence-level matches but not person-level ones, it may just be that this population isn’t present in FIG. That’s a data coverage limitation, not a system failure.
Why identity resolution is crucial for predictive modeling
Your predictive model learns from the signals in your data. The richer the signals, the more precise the model. If we can’t resolve identities, we can’t generate signals. And that means the model is essentially working with blind spots.
Match rate isn’t just a technical stat—it’s the foundation for how much value you can drive with AI. So if your match rate looks low, let’s dig in together. Because the better we know your audience, the better your predictions will be.
Wrapping it all up
At the end of the day, your predictive model is only as strong as the signals it’s trained on. And assuming we’re not talking about limited, first-party only modeling, those signals depend entirely on whether we can recognize the people in your data—and enrich those records with meaningful traits.
That’s why identity resolution isn’t just a behind-the-scenes technical step. It’s the bridge between your raw data and the actionable insights that drive real outcomes. From expanding your audience and overcoming cold start challenges to increasing model precision and campaign ROI, enrichment through the Faraday Identity Graph is what unlocks the full power of predictive AI.
So if you’re seeing lower-than-expected match rates, or if you’re unsure whether your data is giving you the best possible results, let’s take a look together. Fixing a match rate issue might be as simple as a formatting tweak—or it might point to a strategic opportunity to boost your reach and improve your outcomes.
Because predictive modeling shouldn’t just be about what has worked before—it should be about what’s going to work next. And that starts with knowing who you’re modeling.
Ready for easy AI agents?
Skip the struggle and focus on your downstream application. We have built-in sample data so you can get started without sharing yours.