How one subscription box brand leveled up their product recommendations with Faraday’s AI agents
A leading subscription box company replaced manual product rules with Faraday’s AI-powered recommendations—boosting revenue and retention through smarter, scalable personalization.
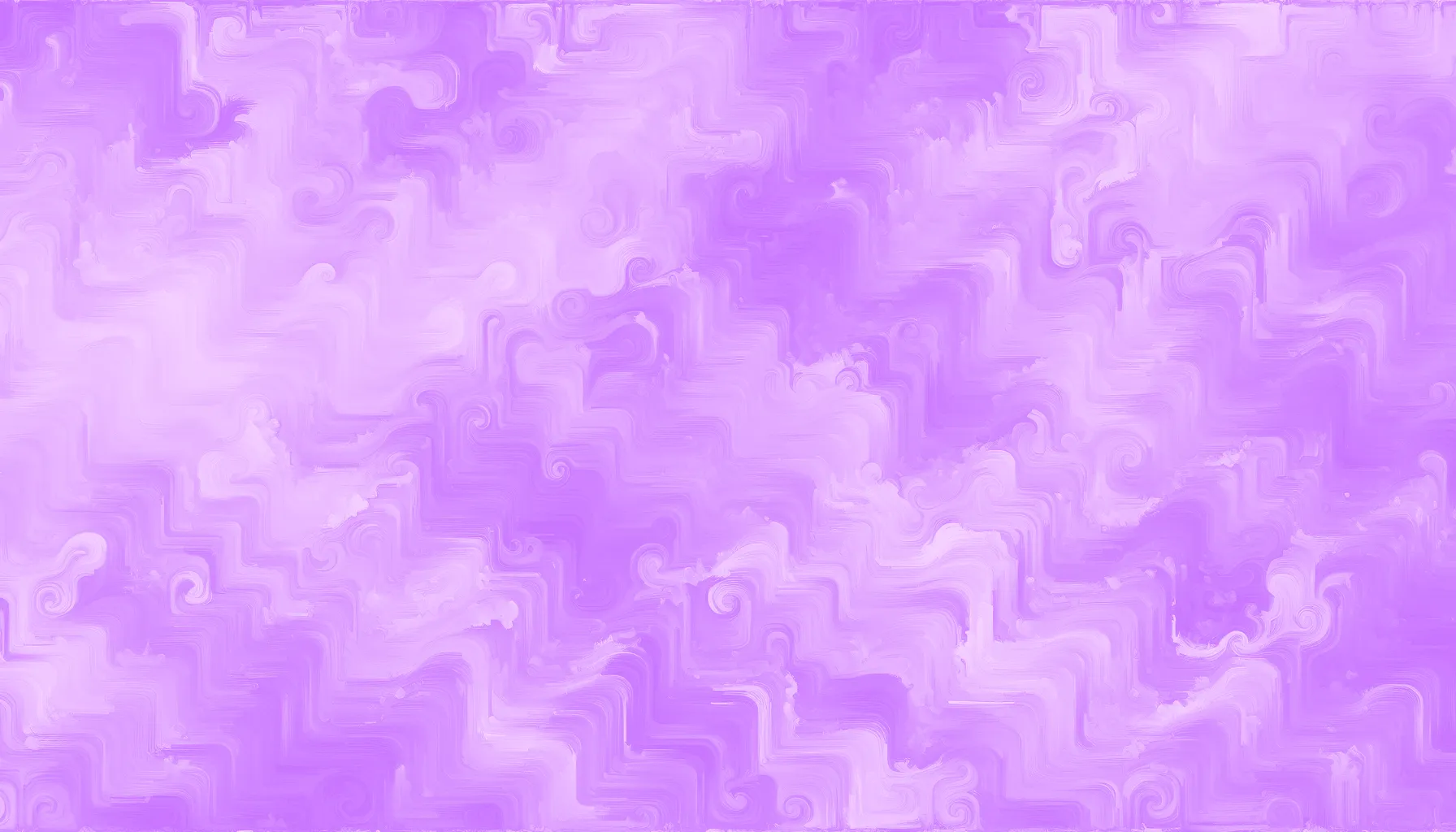
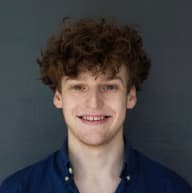
Product recommendation engines are everywhere these days—from Netflix queues to Amazon homepages. But in subscription commerce, where customers commit to recurring deliveries, recommenders aren’t just nice to have—they’re essential. Sending the wrong product doesn’t just hurt engagement. It increases churn, reduces LTV, and erodes trust in the brand.
And that’s why a leading subscription box company set out to improve how they matched products to people, and to solve this problem, they of course came to Faraday first.
The problem: Manual rules and missed opportunities
Before working with Faraday, this brand was manually assigning monthly boxes using basic written rules. While the logic was informed by customer traits and preferences, it was still deterministic, rigid, and prone to human bias. It also couldn’t scale effectively across an ever-growing catalog or subscriber base.
Most importantly, the team didn’t have a reliable way to test whether these rules were actually the best way to drive retention and increase revenue.
The solution: AI-powered product recommendations
To modernize their process, the brand partnered with Faraday to implement a machine learning–based recommendation engine. Unlike traditional scoring models that evaluate products in isolation, Faraday uses a two-sided matching framework—scoring both:
- Customer affinity: How likely a specific person is to engage with a product
- Product performance: How well that product has performed with similar audiences
This leads to smarter, high-confidence pairings optimized for both personalization and conversion—at scale. With Faraday, the brand was able to deliver more relevant recommendations, reduce bias, and adapt to new products and preferences as their catalog evolved.
The results: Higher revenue and lower churn
The team ran a clean A/B test comparing Faraday’s algorithmic recommendations to their manual rules.
The outcome:
- 📈 ~5% initial lift in revenue per customer
- 📉 ~3% reduction to churn rate
- 💰 Over 5x ROI generated monthly with Faraday
- 🗓️ Four consecutive months of our recommendations outperforming their rules-based alternatives
These weren’t marginal gains—they were statistically significant improvements that validated the shift toward AI. And what’s more, this is just the start. The more this brand uses our models, the more they’ll learn and the better they’ll get, meaning they can expect even better results over time.
How Faraday recommendations work
Faraday’s recommender system doesn’t just surface “popular” products. It predicts the mutual affinity between a person and a product, using thousands of behavioral signals—purchase history, tenure, geography, psychographics, and more. It also incorporates product-level attributes and past performance to ensure the best-fit items rise to the top.
What’s more, clients can tune the system with constraints like inventory availability, product diversity, or subscription cadence—ensuring not just personalization, but operational feasibility.
In conclusion
Want to see how our recommenders could work for your business? Just reach out and talk to sales, they’ll tell you exactly how this use case, as well as a range of other AI agents could help your business thrive!
Ready for easy AI agents?
Skip the struggle and focus on your downstream application. We have built-in sample data so you can get started without sharing yours.