Predicting a customer’s propensity to buy using machine learning
Using propensity to buy models to target your most likely-to-buy customers is an attractive prospect, but it can be a long, resource-draining process. Read about how customer prediction platforms can drastically shorten your time-to-value.
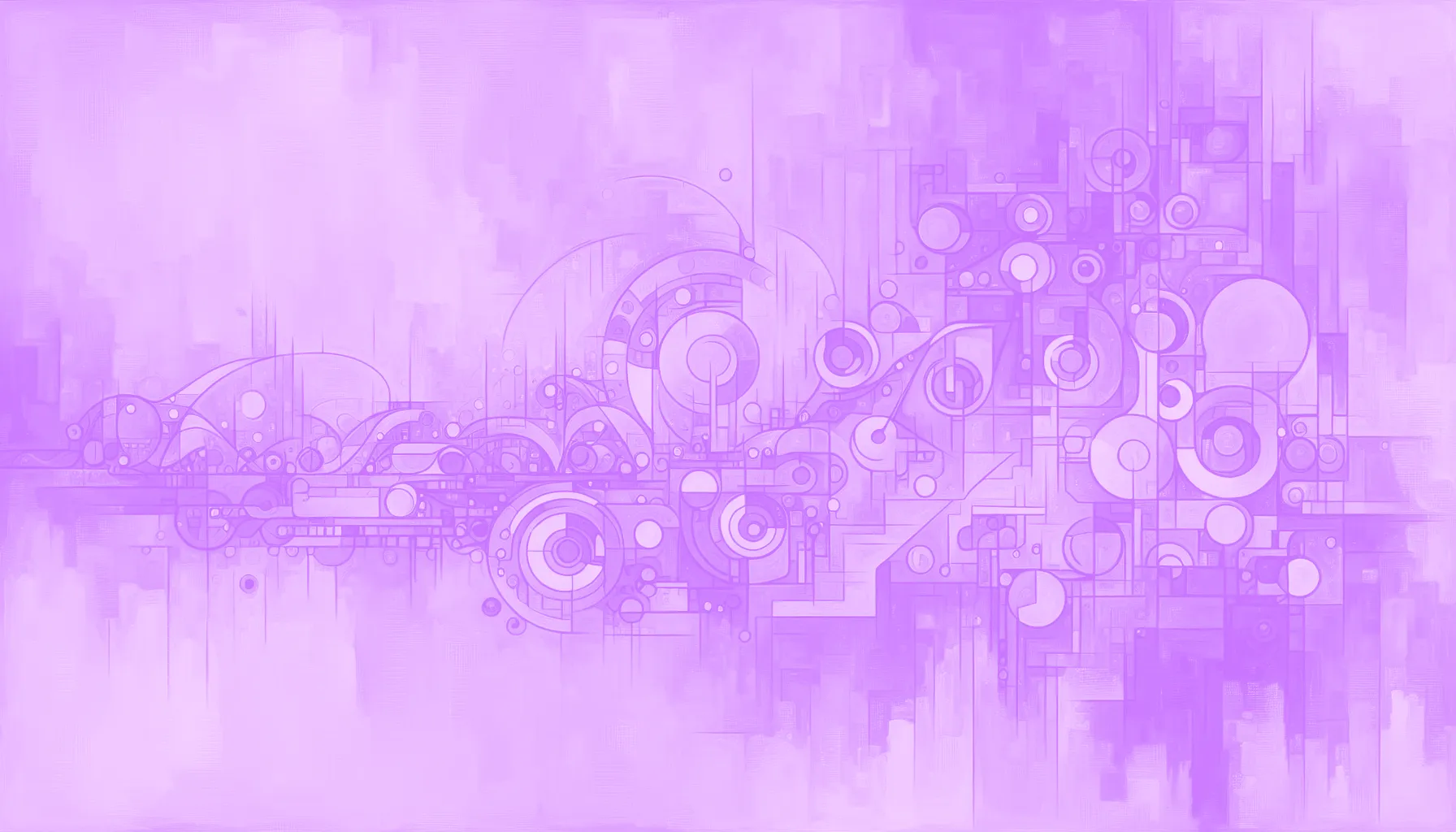
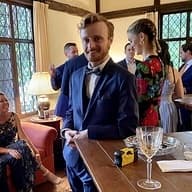
These days, marketers are looking for every advantage to help them stand out from the crowd. From personalization to utilizing AI tools like ChatGPT to lend a hand with copy, consumers are getting more picky about which brands they interact with, and it’s forcing brands to adapt. One such advantage makes use of predictive analytics and machine learning to enable brands to stay one step ahead of their customers and their competition: propensity to buy.
What is propensity to buy?
It’s likely that either you or someone on your team has found themselves muttering “I wish I knew whether or not this lead is going to be a waste of time,” or “if only I knew this customer was about to churn” at some point recently. Predicting propensity to buy–or propensity, in short–is using a machine learning algorithm like random decision forest to predict how likely someone is to take an action. And it doesn’t just apply to new customer acquisition, or even just lead conversion. You can predict the propensity for virtually any action, meaning that your sales and marketing tactics become proactive rather than reactive.
Benefits of propensity to buy
Propensity to buy models can be applied to an incredible variety of use cases that provide real, actionable insights for the modern marketer. Let’s take a look at three.
Market sizing
Expanding your brand’s reach into a new territory is inherently risky. Manual market sizing analysis can help mitigate the risk, and smaller campaigns can test the waters and see if there are any bites from your ideal customers, but then you’re spending both time–your most valuable resource–and money, on a strategy that may or may not pan out. Sure, brands have been doing that forever–but with propensity to buy models removing the guesswork in a market sizing analysis, it can be done accurately and with confidence.
Ad targeting
Similarly, propensity to buy models can be applied across the entire U.S. population, providing likely buyer scores that let you easily filter out the bad fits. By eliminating the bad fits that were never going to buy in the first place, the extra spend that would’ve gone toward showing them ads that weren’t relevant can go toward ones that resonate perfectly with your ideal customers.
Retention campaigns
The effectiveness of propensity to buy extends beyond acquisition campaigns–they’re equally as important and effective for retention as well, through propensity to churn models. The dream of knowing that a customer’s going to churn before they make that critical decision becomes a reality through actionable churn scores. The higher the score, the more likely they are to churn, giving your team crucial insight and warning to keep them on board through a discount, promo offer, or some other attractive deal.
How do propensity models work?
Let’s take a look under the hood: how does it work? Propensity models use machine learning algorithms to pore over your customer data to find historical patterns in it. Customers placing orders, churning, ordering again, and more are compared and analyzed to compare each person to another for any similarities. Often utilizing algorithms like random decision forests or logistic regressions, propensity to buy models–while data-hungry, resource-intensive, and time-consuming–offer an attractive variety of impactful use cases.
How to build propensity to buy models
You can build models to predict propensity to buy and use those scores directly in your stack to gain the benefits that come with knowing when a lead is likely to convert, a customer churn, or some other use case. Let’s dig into some key aspects of building propensity to buy into your stack.
A strong foundation of data
Data is the most important feature of propensity to buy and customer predictions. Without a strong enough foundation, the predictive models that your data science team builds won’t have enough data to predict strongly off of, leaving you with questionable-at-best insights. You’ll want a strong mix of first-and-third-party data, and that data should include demographics, psychographics, lifestyle, property, financial, and more so that your data is well-rounded.
Data science to build predictive models
With your data locked in, you need data scientists to build predictive models. Regardless of which machine learning algorithms your team uses, they’ll need time to build the models and for the models to iterate–machine learning needs time to learn the data that it’s working with, and will grow smarter the longer the algorithms run. This often takes anywhere from 5 months to over a year.
Systems integration to fit in your stack
The last major pillar of building propensity to buy models is finding a place for it in your stack. You can have propensity to buy scores sent directly to your database or warehouse, to your CRM, or any other activation platform. Depending on where you decide to implement, you’ll need to build an integration of some kind that enables your workflows to continue uninterrupted–a process that can take several weeks to complete.
How Faraday can help implement propensity to buy in your stack
Faraday is purpose-built to cut the build-it-yourself timeline of building propensity to buy down from monthslong down to mere days. With Faraday, your brand has access to built-in, cookieless, permissioned consumer data on nearly every U.S. adult, providing rich insight into your customers, and a data science team that has been at it for over a decade. Propensity scores for any action your brand is interested in can be embedded anywhere in your stack through hundreds of pre-built integrations to place your predictions exactly where you need them.
Predict propensity to buy to stay proactive
Make use of Faraday’s propensity models to predict–in just a few clicks–who’s (or where’s) most likely to buy, who’s likely to churn, what leads are most likely to convert, & more. Propensity to buy models with machine learning make your brand proactive so that you’re always delivering the right message at the right time.
Ready to try it out? Create a free account.
Ready for easy AI agents?
Skip the struggle and focus on your downstream application. We have built-in sample data so you can get started without sharing yours.