Predictive modeling generates tangible value, and we can prove it
Faraday uses holdout testing to validate predictive models before you commit, ensuring they deliver real, measurable results.
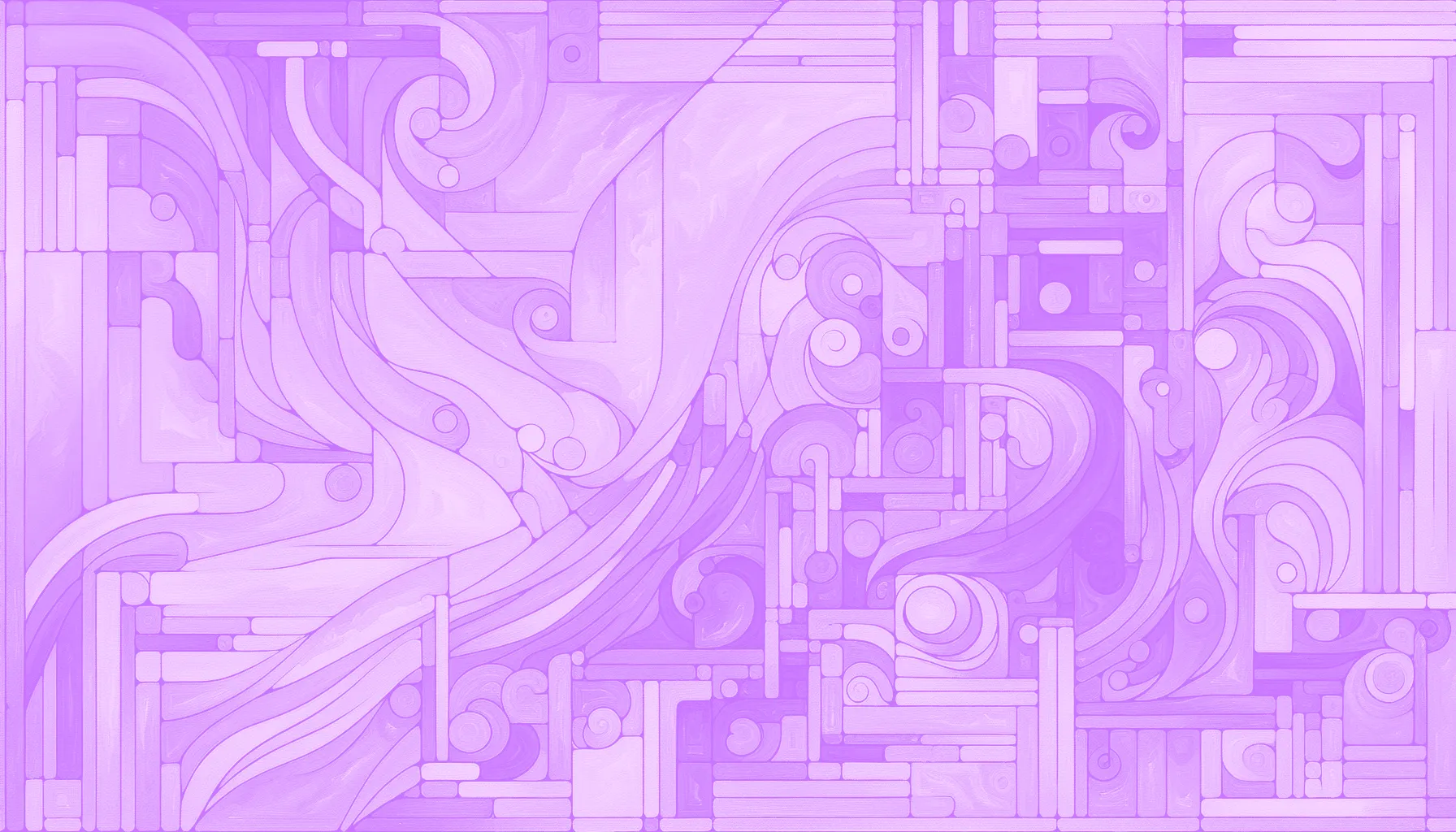
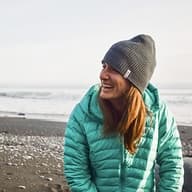
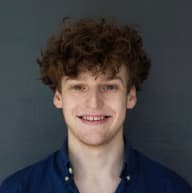
If you are reading this, you are probably talking to Faraday about a potential contract and have a healthy amount of skepticism as to whether we can actually do what we say we are going to do.
And here’s the thing: you’re right to be skeptical. With tight budgets and increasing pressure to show ROI, you can't afford to bet on unproven solutions or spend countless cycles figuring out if a tool is really going to live up to the promise. You need a way to separate legitimate predictive tools from the noise.
Every week, we get to talk to dozens of CMOs, CTOs, & CEOs across industries who are trying to discern the same thing: which tools are legitimate and which ones are not. As a leader of a high profile brand, you are inundated with promises of predictive insights and transformation, but you need more than just promises – you need guarantees.
That's where our holdout evaluation comes in. It's not a proprietary methodology we invented – it's an industry-standard approach used by data scientists to validate predictive models.
What makes us different is simple: we run this validation before you sign any contract. Because frankly, if we can't prove we'll deliver real results for your business, we don't want to waste your time.
Below we’ve outlined what we are doing “behind the scenes” and how you can evaluate if we will add the value you need.
What is a holdout evaluation?
A holdout evaluation is a method for validating predictive models by comparing their predictions to real-world outcomes. We set aside a portion of recent conversion data—data the model has never seen before—and use it as an independent benchmark, against which predictions can be compared.
This “holdout set” acts as a real-world proving ground. It is untouched until after the predictive model has been trained.
Meanwhile, the rest of your historical data is combined with rich third-party insights from the Faraday Identity Graph (which includes 1,500 attributes on 240M+ U.S. consumers and their households). We use this combined data to train the new predictive model.
Training a predictive model simply means finding patterns in conversion behavior. In other words, what can we learn from your previous customers to identify future customer behavior? Once trained, the model generates predictions—scoring leads based on their likelihood to convert and only after these predictions are made do we compare them to the real conversion rates in the holdout set.
If the highest-scoring leads actually convert at higher rates than lower-scoring ones, the model is validated as accurate and reliable.
Holdout evaluations ensure predictive models aren’t just theoretical—they work in the real world, using real data, to deliver real results.
How holdout validation works at Faraday
We've streamlined this validation process to be transparent and straightforward:
-
Prepare your data and set aside a holdout group
We start with your recent lead conversion data. Before any processing occurs, we reserve a portion of your most recent leads as a "holdout set"—data that the model will never see during training. This ensures we have an independent benchmark for evaluating predictions.
-
Combine data with Faraday Identity Graph
With the holdout set reserved, we then combine remaining data with Faraday’s built-in, rich consumer data sourced from the Faraday Identity Graph. This expanded dataset provides a deeper view into key predictive signals, like property and demographic information, life events, buying habits, and more.
-
Train the model and begin predictions
Using this enriched dataset (excluding the holdout set), we train a predictive model to identify patterns in conversion behavior—learning from traits, behaviors, and other signals.
Once the model is trained, it scores each lead based on its likelihood to convert, generating a ranked list of opportunities—from high-value leads to those less likely to convert.
-
Compare predictions to reality to validate model
Finally, we put the model to the test: we compare its predictions against the real outcomes in that untouched holdout set. If the highest-scoring leads don't convert at significantly higher rates, we go back to the drawing board.
How to know if a holdout validation was successful
A holdout validation is considered successful when the following criteria are met:
-
Clear correlation between predicted probability and actual conversion rates
The model’s predicted likelihood to convert should directly align with the actual conversion rates. If the predictions are accurate, high-probability leads should convert at a noticeably higher rate than lower-probability ones. -
High-scoring leads convert at significantly higher rates
The top-scoring leads should consistently perform better than those ranked lower. This demonstrates that the model is identifying truly valuable leads. -
Consistent performance across different customer segments
The model should show reliable results across various segments—whether you’re targeting different demographics, regions, or other customer profiles. If the model works well across a broad set of data, it proves its reliability and scalability.
When a model meets these criteria, it’s ready for deployment—whether that means optimizing lead buying, refining your direct mail strategy, or enhancing your email personalization approach.
Questions you should ask us
We want you to push us on what matters most for your business. Here are key questions to evaluate if we're the right fit:
-
How does this model's performance compare to the lift you are seeing with similar businesses in our industry?
While we can’t share client specifics, we can provide insight into broader trends and expected performance benchmarks.
→ Why this matters: Understanding industry benchmarks helps you gauge whether predictive modeling is truly a competitive advantage for your business.
-
How much historical data do we need for reliable predictions?
The answer varies by business model and conversion volume. We’ll help determine whether you have enough data—or how to supplement it if needed.
→ Why this matters: Predictive models work best when trained on strong data. Knowing the threshold for reliability ensures you're making data-driven decisions with confidence.
-
What happens if the model doesn't perform as expected?
We have a structured iteration process to refine models when necessary, ensuring they reach meaningful predictive power.
→ Why this matters: No model is perfect on the first run. Understanding the process for continuous improvement ensures long-term success.
-
How will this integrate with our existing marketing stack?
We’ll provide technical specs, discuss implementation timelines, and outline the resources needed to get up and running.
→ Why this matters: Seamless integration means faster time-to-value and less friction in your workflow.
Why holdout evaluations work
The businesses who win this year will be those who successfully incorporate predictive modeling into their core workflows. It's no longer a nice-to-have – it's a need-to-have. But we know you can't afford to make decisions based on hunches or unproven tools. That's why we're eager to validate our value up front.
At the end of the day, three things matter to us:
-
Confidence before commitment:
You see proof before you commit -
Higher marketing efficiency: Your marketing dollars go to the leads most likely to convert
-
Stronger sales performance: Your sales team focuses on opportunities worth their time
If we can't demonstrate value, we'll be the first to tell you we're not the right fit. But if we can – and we usually do – we want you to know the exact savings or additional revenue you can expect to see over the course of the next year with Faraday. Interesting in generating value you can trust? Reach out to learn more about what we can do for your business!
Ready for easy AI?
Skip the ML struggle and focus on your downstream application. We have built-in sample data so you can get started without sharing yours.