The modern marketer’s guide to personalization at scale
Consumers are demanding personalized content at an increasing rate, but personalization at scale has traditionally been out of reach for many brands. Using a customer insights platform makes true personalization at scale more accessible than ever.
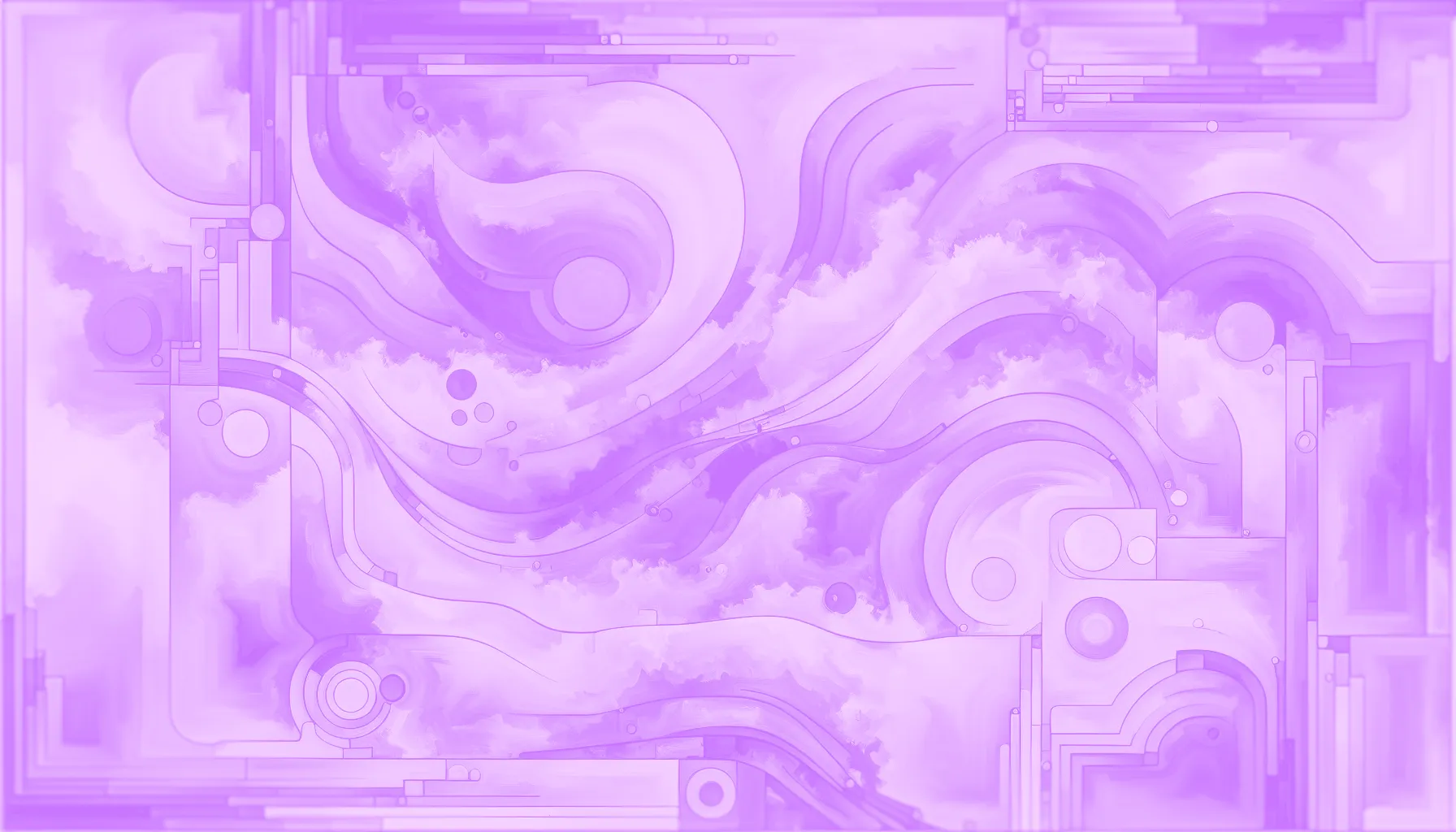
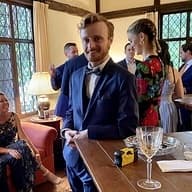
Personalization is the holy grail of growth teams everywhere. At this point, everyone knows how important it is to speak to their customers and prospects like they’re real people, and not just orders and clickstreams. It’s often easier said than done, though, and brands with a significant amount of data and a large customer base may find themselves struggling to make heads or tails of how to organize it, or even know what data is worth focusing on.
Challenges of personalization at scale
Doing personalization at scale right can provide incredible value to your brand, and has a major impact on how your customers see and interact with you. A daunting set of challenges is presented to most brands that try to tackle the issue themselves–let’s dive into a few of the most glaring challenges.
Data, and a lot of it
To know more about–and how to speak to–your customers, you need to have data on them. Through the data you currently have, you’ll likely have some level of insight into the demographic, financial, and transactional background of your customer base as a whole. Though this data is strong, and gives a unique perspective due to it being exclusive to your brand, your customers exist outside of interacting with your brand. A healthy mix of first-and-third-party data can provide additional layers of complexity and nuance to how you view your customers–and how you engage with them.
The problem is that you need a lot of strong data to personalize meaningfully, and the more data you collect, the harder it becomes to personalize at all.
Finding the relevant data
Collecting the data is the first step to creating an impactful strategy for personalization at scale–but what do you do with it? Historically, data-savvy marketers have sifted through the terabytes of data they’ve collected to try and piece together what makes up their ideal customer, and what their key levers are. If your brand is thorough, this is a ton of data to sift through, and the process of finding what’s relevant–on the whole as well as on the individual level–is colossal.
Personalization at scale
By using machine learning for predictive personalization, the effort involved in scaling personalization across your entire customer base–past, present, and future–isn’t as herculean as it sounds. Machine learning algorithms can segment your customers into bespoke, cohesive personas that you can deploy anywhere in your stack, allowing you to create individualized, personalized campaigns for any given subgroup of people you’re interested in.
Responsibly-sourced data
Privacy is front-and-center of any data-focused discussion in 2025–as it should be. It began with the (albeit slow-rolling) death of third-party cookies, and will continue to be a primary focus of consumers moving into the future. Privacy matters, and largely, people are uncomfortable with things like cookies–previously critical to a third-party data strategy–tracking them creepily across the web to find out what they’re searching and where they’re visiting, even if the end-result is personalized content.
The data you collect and use should be responsibly-sourced, opt-in, and permissioned. It’s possible to provide a perfectly personalized experience while simultaneously being a privacy-forward brand, but it takes effort.
Benefits of personalization at scale
With the barriers to true personalization at scale being so high, is the end-result worth it? With companies that personalize collecting 40% more revenue than those that don’t, the answer is a resounding yes.
The right message, at the right time, every time
It’s an adage as old as marketing itself, but it’s always going to be true when a single poorly-personalized, or otherwise irrelevant message could mean the loss of a customer–or potential customer–forever. With personalized content delivered to every individual you’re engaging with, at any scale of your campaign, you’ll always be delivering the right message, at the right time, every time.
Consumers demand personalized content
With 61% of consumers reporting that they feel that most companies treat them as a number rather than a person, it’s clear that personalized content is near and dear to the modern consumer’s heart. As a dog lover, you wouldn’t want to receive a postcard from a pet food brand focused exclusively on cats, would you? Your customers don’t either.
Building brand loyalty
78% of consumers say that they’re more likely to buy again from brands that personalize their content. Loyal customers are also more likely to recommend brands they love to their friends & family, ensuring that, if you focus on keeping your customers happy, they’ll return the favor by shouting your brand’s name from the rooftops. Keeping a strong base of loyal customers is key to a good churn prevention strategy.
How to achieve personalization at scale
It’s clear that the benefits of personalization outweigh the challenges, but how exactly can it be achieved? There are three key pillars to successful personalization at scale, and they all focus on your customer data.
Collect good first-party data
Data is the most important feature of propensity to buy and customer predictions. Without a strong enough foundation, the predictive models that your data science team builds won’t have enough data to predict strongly off of, leaving you with questionable-at-best insights. You’ll want a strong mix of first-and-third-party data collected through tactics like web forms, surveys, and loyalty programs, where you can really draw out what makes your customers unique.
Optimize your data with first-party data enrichment
As good as your own customer data is, your customers have more going on in their lives than you can see through their interactions with your brand. You can buy data from companies like Acxiom or Epsilon, who have been collecting data for years and years, or you can leverage assets like publicly available census data to find more about who your customers really are.
Use AI for customer segmentation
With your data collected and enriched, you need to segment your customers into unique subgroups, or personas. It can be manually done, but the process is incredibly labor-and-time-intensive, and is prone to human error. Using AI for customer segmentation takes the load off of your team, leaving you ready to personalize campaigns at any scale, create internal, persona-based talk track alignment, and more.
How Faraday supports personalization at scale
Faraday lets you easily identify distinct personas and predict customer behavior with machine learning, unlocking powerful insights to shape better personalization strategies at scale.
Unified, responsible data
Faraday unifies your first party customer data with detailed, opt-in, permissioned, cookieless consumer data, giving you the insights your brand needs while simultaneously being privacy-centric. Hundreds of datapoints through demographics, psychographics, financials, property information, and more to be leveraged for your customer predictions.
Consumers have adapting needs, and 65% of consumers expect brands to adapt to them. This is a laborious task manually, but with Faraday’s built-in data always staying up-to-date, your brand can adapt alongside your customers.
Delivering personalized messaging and creative across multiple channels and touchpoints
Faraday takes your data–combined with Faraday’s–and uses AI to segment your customers into unique subgroups, or personas, based on hundreds of datapoints.
With your customer personas predicted, easily kick off campaigns by deploying your entire persona set, or a single persona, to anywhere in your stack. Every individual your brand is interested in, revealed with clear-cut traits like dog owner, grouped with people that share similar interests, shopping behaviors, and more.
Personalization at scale has never been easier, or more accessible.
Predicting the next best product for every individual
Alongside your customer personas, you can optionally predict the propensity, or likelihood, that any action is taken by an individual. For example, take your Emerging Adult Eli customer persona and predict the likelihood that anyone in that persona will repeat purchase, and, further, predict the next best product for them based on similar shoppers.
Providing personalized discounts to foster customer loyalty
Personalization extends beyond the copy and creative that’s in your engagement with a given customer–it can include providing strategic discounts to customers that have, for example, made a second or third purchase. This way, your customer predictions guide you toward the people that are most likely to repeat purchase so that you can then turn around and give them just the right discount they need to keep coming back.
Ready to achieve true personalization at scale? Create a free account.
Ready for easy AI agents?
Skip the struggle and focus on your downstream application. We have built-in sample data so you can get started without sharing yours.