How Marley Spoon predicted churn 5X better with machine learning
Learn how the meal delivery subscription brand was able to identify likely churners 5X better with machine learning predictions.
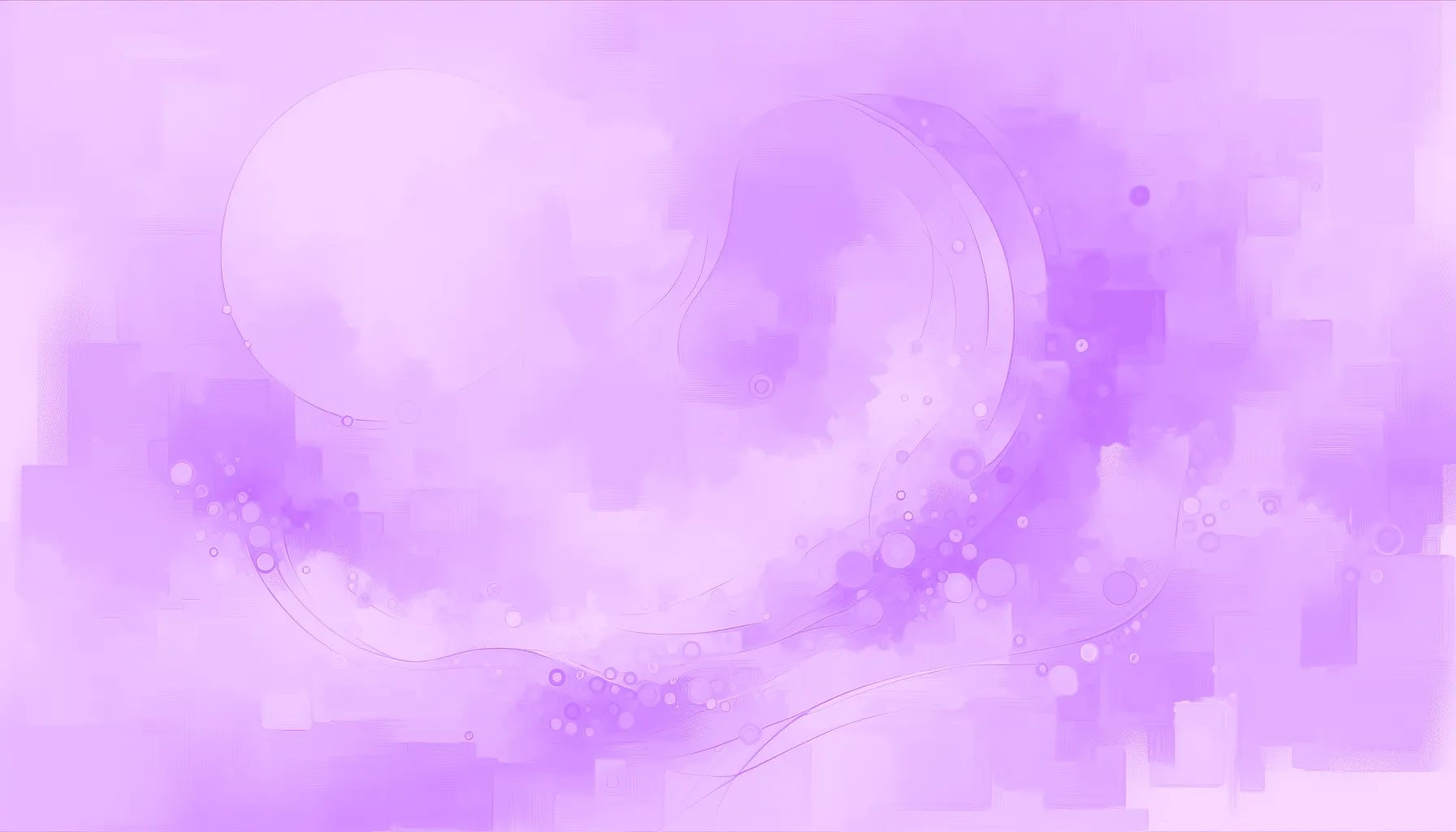
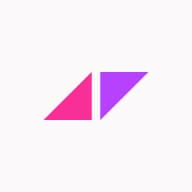
Like many subscription services, meal delivery brand Marley Spoon faced the challenge of retaining customers. High churn rates made it difficult to cover acquisition costs and scale revenue, so they needed a way to identify risky customers. To improve their retention capabilities, Marley Spoon approached Faraday to assess the predictive accuracy between traditional segmentation and AI-driven churn modeling techniques.
Traditional segmentation vs machine learning
With traditional methods, brands analyze trends among customer segments and take action to prevent those with high churn rates from unsubscribing. This is a relatively reactionary measure. In contrast, leveraging machine learning models to score leads and customers for their propensity to churn allows brands to segment customers based on churn risk and properly engage high-risk customers before they unsubscribe.
Analysis and results
Because we’re most interested in the models’ ability to accurately predict churn, we evaluate performance based on how many churned customers were included in the top 10% and top 20% of predicted churners. Here are the results:
Churn rate comparison:
- 19% churn rate for likely churners identified with Faraday churn predictions
- 12% churn rate for likely churners identified through traditional segmentation
Churn detection lift:
- 70% lift over baseline with Faraday churn predictions
- 15% lift over baseline with traditional segmentation
To sum it up, Faraday's machine learning predictions detected churn 5X better than the traditional technique.
Ready for easy AI agents?
Skip the struggle and focus on your downstream application. We have built-in sample data so you can get started without sharing yours.