Data science + data engineering: How Faraday’s forward-deployed team fulfills all your data needs
Faraday’s forward-deployed data scientists and engineers work directly with clients to ensure clean, structured data and precise predictive models—maximizing accuracy, efficiency, and business impact.
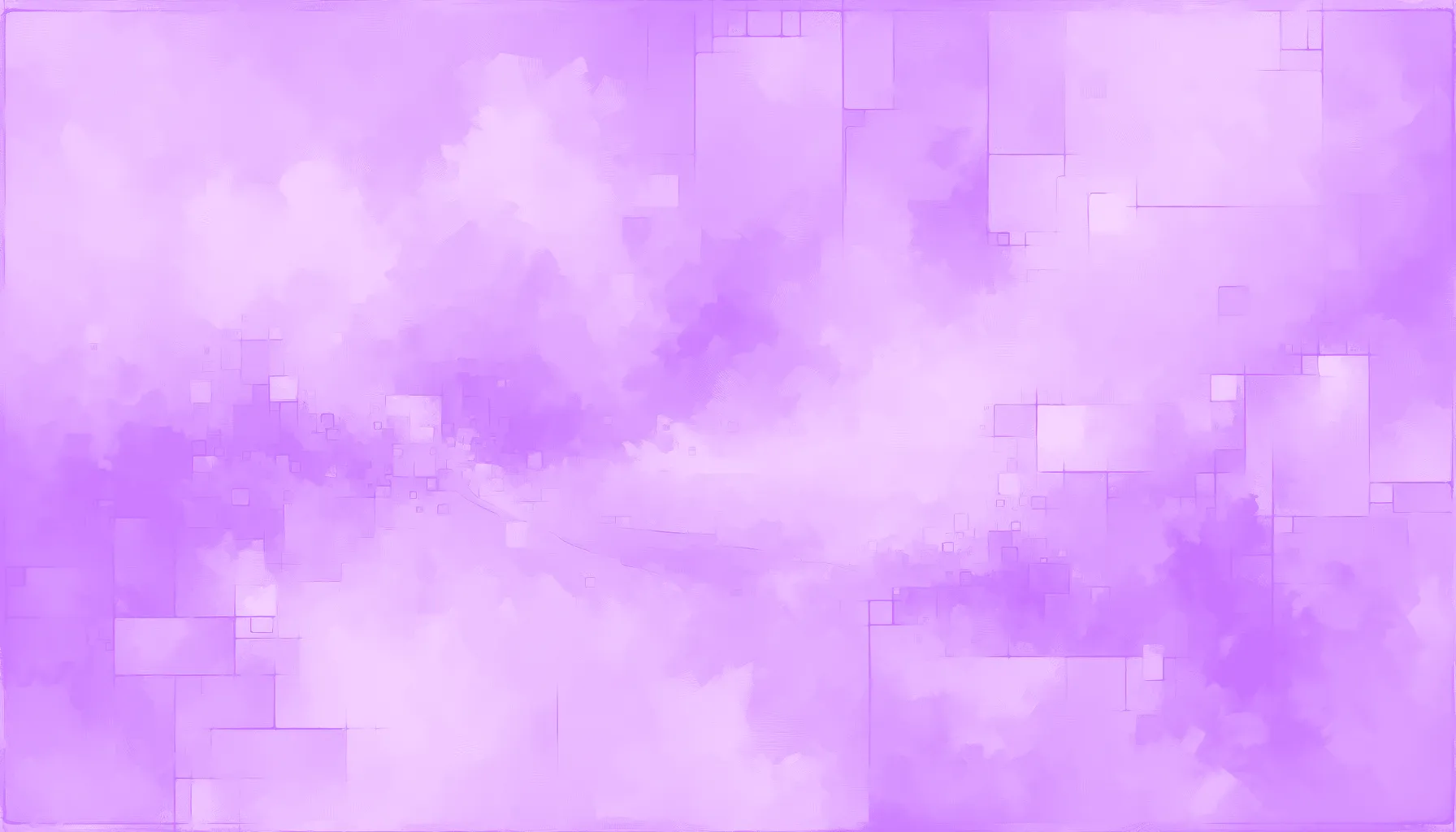
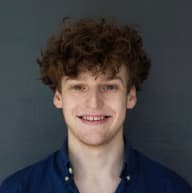
This post is part of a series called Getting started with Faraday that helps to familiarize Faraday users with the platform
At Faraday, we talk a lot about Forward-Deployed Data Science (FDS)—our team of data scientists who work directly with clients to build predictive models, validate results, and ensure you get the most out of AI agents. This service (although new) has already proven invaluable to our clients. It means that they get not only accurate predictions, but comprehensive strategic plans to ensure that our agents actually drive business value.
But data science is only half of the equation. The best predictive AI agents also require another type of data specialist to operate at maximum efficiency: Data Engineers.
And just like our data science team, Faraday’s data engineers don’t disappear behind the curtain—as is often the case at our competitors. Instead they work directly with clients, in a “forward-deployed” capacity. They’re the ones helping you format and structure your data correctly, making sure your onboarding process is as smooth as possible, and reducing or resolving errors to ensure your pipeline stays live and healthy. If you’ve ever submitted a dataset and gotten feedback on how to improve it, or had someone help troubleshoot an ingestion issue, you’ve already worked with our data engineering team.
Take Chris Thomas, one of our incredible data engineers, who coincidentally just wrote a whole blog about how to best avoid pitfalls while importing your data into Faraday. His job—and the job of all our data engineers—is to make sure your data is clean, structured, and ready for predictive modeling. And in just the last weeks he’s helped numerous clients avoid pitfalls that could have set their timelines back by months—thanks Chris!
So what’s the difference between data science and data engineering, and how do both help you get the most out of Faraday? Let’s break it down like Raygun!
Data science: turning data into predictions
Forward-Deployed Data Scientists (FDS) focus on understanding your business goals and applying AI to help you achieve them. They understand the nuances and behaviors of the predictive AI agents that power our business, but also can provide strategic advice on the implementation of agents that ensures that your predictions drive value.
Their job is to help you:
- Define and refine your use case—what do you want to predict? How can AI drive better decisions for your team?
- Data discovery and solicitation–what data is needed for the use case.
- Build predictive models that identify key audiences, behaviors, and trends.
- Validate model performance to ensure accuracy and impact before deployment.
- Interpret and apply insights so you can confidently take action based on data-driven predictions.
For example, if you’re a solar company looking to expand into new markets, an FDS won’t just give you a model—they’ll help you determine the right questions to ask in the first place. How do we ensure that the data used for training isn’t biased by work in existing markets? What is the logical cut off for differentiating promising new markets from a likely low performing market? Which of our powerful AI agents can increase your conversion rates the fastest? By framing the problem strategically, they ensure that your predictions are aligned with real business objectives—helping you make informed decisions that drive sales, optimize marketing spend, and accelerate growth.
Data engineering: optimizing your data and models
Data Engineers on the other hand focus on the infrastructure that makes data science possible, while working directly with you to ensure your data is in top shape.
Their job is to help you:
-
Structure your data correctly before ingestion so it matches well against the Faraday Identity Graph (FIG).
-
Troubleshoot data issues so you don’t lose time on formatting errors.
-
Automate through properly configured integration so your data flows seamlessly into and out of Faraday without constant manual updates.
Where data science can be viewed as more strategic and high-level—focusing on where you want to go, why, and how it will affect your business—data engineering is tactical, focused on how to get there efficiently. Engineers ask questions like, “How can we best implement this idea?” or “What changes will make this process more efficient?”
At Faraday, a Forward-Deployed Engineer (FDE) like Chris undertakes tasks like supporting integrations with your data warehouse, reviewing your data during ingress, flagging potential issues, and suggesting optimizations to improve matching and model performance.
At Faraday, you benefit from both types of data experts
We don’t offer both Forward-Deployed Data Science and Forward-Deployed Data Engineering just because it sounds nice (although doesn’t it?)—we do it because it’s the fastest way to get actionable insights into our clients’ hands.
Making data engineering a client-facing function is a huge step that moves the process forward, ensuring that costly holdups and mistakes don’t happen before your predictive models even have a chance to work and that they keep working at peak efficiency after deployment.
Here’s what that means for you:
🤔 No more guessing if your data is good enough. FDEs provide hands-on support before and during ingestion, catching issues early so they don’t slow you down later. After deployment they’re the ones making sure your models stay healthy.
⏳ Faster time to value. Instead of back-and-forth troubleshooting that delays model deployment, our FDEs work with you upfront to get data right the first time.
📊 Stronger, more accurate predictions. The best AI models in the world can’t fix bad data. By optimizing your data at the start, we ensure your models perform at their peak.
💰 Fewer costly mistakes. If your data isn’t structured correctly, you could end up with mismatched records, poor model performance, or wasted budget. FDEs help prevent that before it happens.
🤝 A seamless experience. Whether you’re working with an FDS to interpret predictions or an FDE to streamline integrations, you have direct support from an expert who understands your business.
In short, data science helps you make smarter decisions, and data engineering ensures your data is ready to support them. While forward-deploying both roles isn’t yet standard practice in the industry, we believe transparency, explainability, and speed to deployment aren’t just nice to have—they’re essential. And we’re not in the habit of waiting for the industry to catch up.
Our clients see the impact firsthand. Numerous businesses have leveraged our forward-deployed approach to streamline their data, improve model performance, and drive real results. You can explore a few of their success stories here.
If you’re already working with Faraday, you know what this means for your business and bottom line. And if you’re not—shouldn’t you be?
Let’s chat about how we can help you put your data to work.
Ready for easy AI agents?
Skip the struggle and focus on your downstream application. We have built-in sample data so you can get started without sharing yours.