How this debt consolidation company optimized direct mail with lead suppression to save over $100K each month
Through predictive lead suppression, this company was able to cut at least 600,000 pieces of least-likely-to-convert direct mail each month.
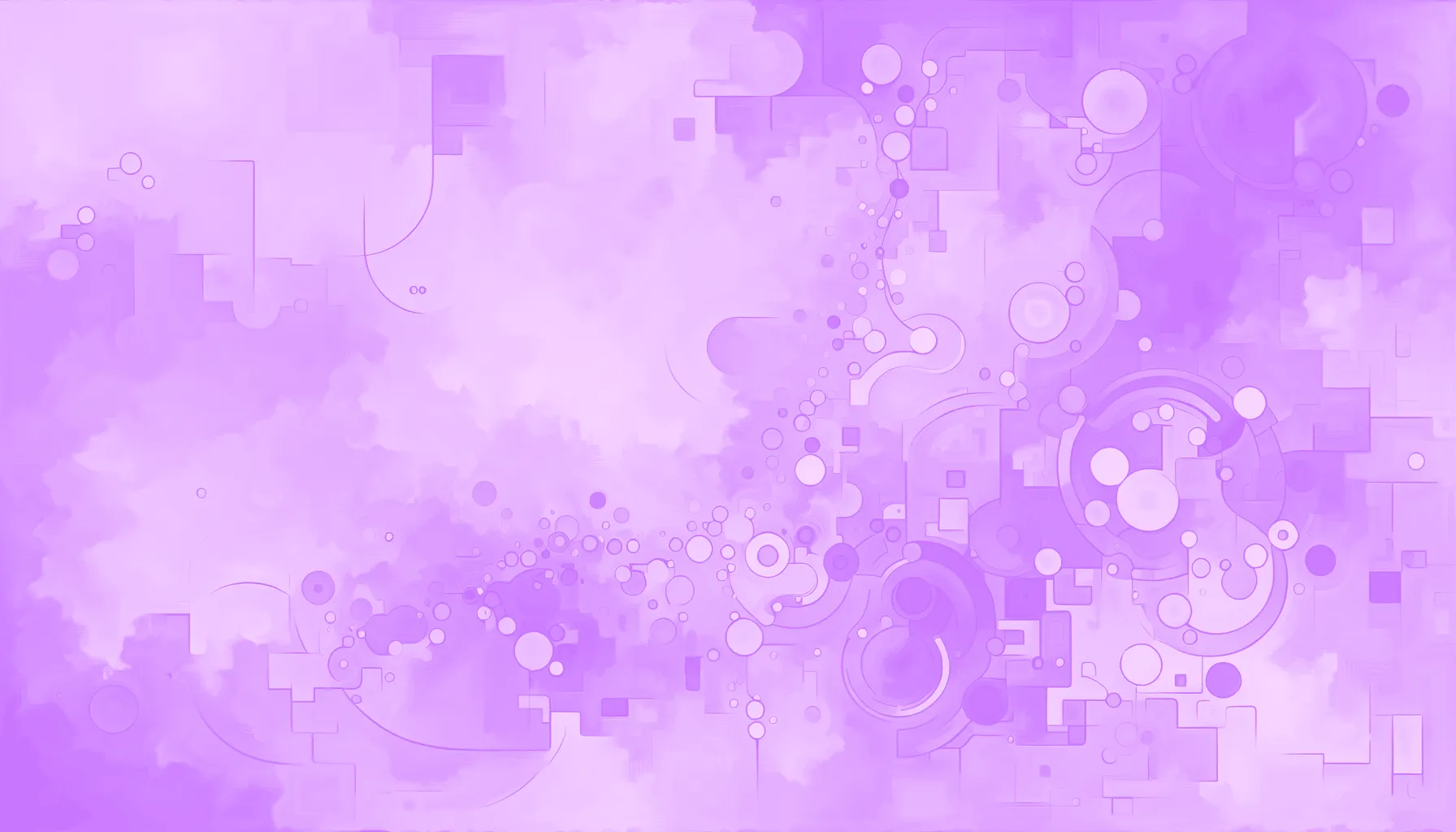
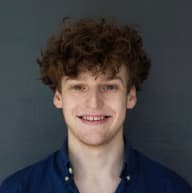
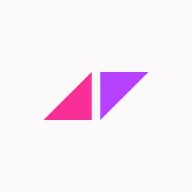
A leading debt consolidation company relied on direct mail as a key channel for acquiring new customers. However, a significant portion of their marketing budget was being spent on sending mail to leads who were unlikely to convert. This inefficiency not only increased costs but also diluted the impact of their outreach efforts, making it difficult to connect with high-intent buyers.
Smarter targeting with Faraday's lead suppression
To address this challenge, the company partnered with Faraday to implement a predictive lead suppression model. This process began with Faraday ingressing their lead data to assess the key characteristics of their existing customer base. Then, through careful analysis of their historical conversion patterns—as well as the inclusion of rich 3rd party data and attributes on 240M+ US consumers that comes preloaded through the Faraday Identity Graph (FIG)—we were able to predict which of their leads were most likely to convert.
Using this analysis, Faraday’s model identified the bottom 10%—the least-likely-to-convert leads—and suppressed them from the company’s direct mail campaigns. This approach immediately reduced wasted marketing spend and allowed the company to reallocate resources toward higher-value prospects.
Or see Faraday's lead suppression process broken down visually
-
Don’t start from scratch
Faraday comes preloaded with 1500 attributes on 240M+ US consumers and their households.
This powerful data set enables us to look at all potential data traits that could impact a consumer’s behavior: property or demographic data, life events, buying habits, etc.
-
Your lead data is shared with Faraday
Your lead data is collected and ingressed into Faraday securely.
This guarantee is achieved through our robust data security policies, which include HIPAA, GDPR, and SOC-2 compliance.
-
We train predictive model by analyzing client data for patterns
Faraday’s Identity Graph & AI/ML predictive modeling enables us to identify unique trends in your data set and specifically assess your leads’ likelihood to convert.
-
We identify how likely each lead is to convert
We identify 10 deciles to be able to help you determine which leads are the best (most likely to convert after receiving mail) and the worst (those that will cost more in postage/print than they will generate in value, or simply won’t convert).
-
We establish a cut-off threshold
Faraday then helps you to calculate the “cut-off” threshold. This determines where the cost to print/send the mailer is greater than the likelihood to convert to revenue.
-
We return the targeted list
Once Faraday has removed the low-fit leads, you can use the new list to target your direct mail process and proceed with your next mail drop.
Optimized results, supported by data scientists
Although this model was already delivering significant value, our data science team wasn’t satisfied.
That was because this company recognized four distinct customer segments within their broader population—each with unique characteristics. Although the one-size-fits-all approach had made sense initially to drive results, we hypothesized that a single model that handled all leads at once was likely not actually able to provide the highest level of precision possible. Instead, we considered creating four additional models which would be individually tailored to each unique customer segment.
While we were confident that this more granular approach would improve results, at Faraday, we prioritize empiricism over intuition so we decided to run rigorous validation tests. And indeed, after comparing conversion trends between the original model alone and all five models together, we were able to prove—rather than assume—the added value of the more granular approach. For the client, this meant even better precision in their targeting and more return on their direct mail spend.
While this process required a deeper investment of our data science team’s time, the outcome was clear: greater accuracy, better optimization, and ultimately, higher value. At Faraday, this isn’t just a one-off added service—it’s how we operate. Strategic data science investment is the only way to unlock insights at this level, and this project stands as a testament to that philosophy.
The result
The impact of Faraday’s predictive lead suppression was immediate and substantial:
- At least 600,000 fewer pieces of wasted direct mail sent to low-intent leads monthly
- Over $100,000 saved each month in direct mail costs
- An additional $20,000 in monthly revenue, or $240,000 annually, thanks to these targeted models’ better precision in predicting which customers would convert
With these enhancements, the company is now seeing a 10x return on investment (ROI) from Faraday’s predictive modeling.
Beyond cost savings: data-driven growth
By investing in Faraday’s predictive modeling and data science expertise, the company didn’t just cut costs—they redefined the way they acquire customers. Suppressing low-intent leads was just the beginning. By refining their approach with segment-specific models, they turned direct mail into a high-precision growth engine, ensuring that every dollar spent reached the right audience.
With this new strategy, the company isn’t just saving money—it’s driving measurable, sustained growth. Faraday’s predictive lead suppression has become a core part of their marketing playbook, proving that the right data science investment doesn’t just optimize campaigns—it transforms businesses.
Ready for easy AI agents?
Skip the struggle and focus on your downstream application. We have built-in sample data so you can get started without sharing yours.