How Faraday's predictive lead scoring helps a national brand identify leads with a 4X conversion rate
A third-party analysis revealed that the conversion rate of leads in the top 10% of lead scores was 4x higher than the brand's baseline conversion rate, confirming Faraday's ability to accurately identify high-potential leads.
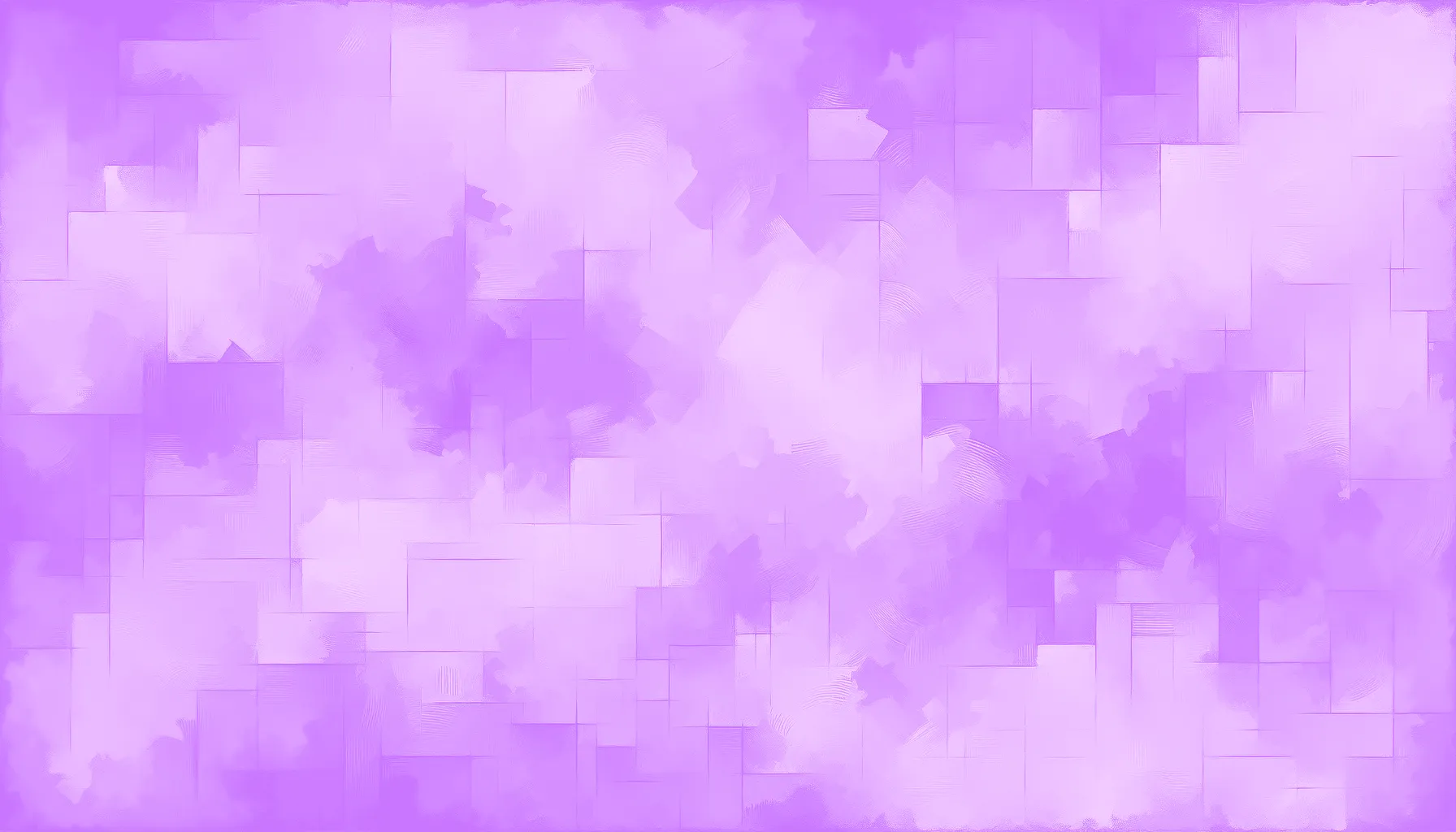
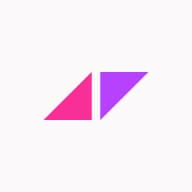
This customer story has been anonymized to respect the company's competitive strategy.
The problem: optimizing their lead scoring methodology
A national home services brand was trying to optimize their lead-to-customer conversion rate. Their existing lead handling methodology was to engage all leads upon entry into their funnel via a call center, then an SMS and email engagement drip for those leads that did not convert. Their active lead engagement window was 19 days from funnel entry to lead conversion. Leads were scored using a rules-based methodology of first-in, first-out with identity verification completed upon form fill completion. Beyond this, all leads were treated the same.
This longer sales cycle resulted in sales representatives having more leads than they could consistently follow up with. The company believed this dynamic was negatively impacting conversion rates with sales reps spending too much time on prospects with a low chance of converting into customers, and too little time on those who were a great fit for their services.
The obstacle: limitations of rules based scoring models
Conventional B2C lead scoring models commonly utilize a rules-based approach, where human judgment determines the rules for scoring leads. Typically, this involves calculating a lead score by establishing rules from a blend of basic demographic, property, or identity details (e.g. owner-occupied household, identity verified, etc.) and observed behaviors such as website visits, email interactions, form completions, and/or call length.
Although these rule-based models aid marketing and sales teams in prioritizing their efforts, they carry notable limitations.
- The criteria for lead scoring in a rules based approach are often subjectively chosen, leading to inaccuracies in assessing a lead's actual potential.
- There is a cold start problem for a new lead that has exhibited little to no behavior. A new lead that has just entered the sales funnel often can't be scored, or the score is inaccurate due to a lack of observed behavior.
- Rules reliant on demographic, property, and/or identity criteria often involve either having to ask the lead for this information, which impacts conversion rates, or licensing 3rd party data, which can be costly and increase a company's privacy compliance and liability burdens.
As a result of these limitations, this national home services team started exploring alternatives to traditional lead scoring.
Why Faraday?
Quantitative rather than subjective lead scoring
Unlike a rule-based lead scoring approach, predictive lead scoring uses machine learning to analyze historical lead data and create statistical models. This approach predicts the likelihood of a lead becoming a customer through quantitative analysis, rather than qualitative assessment.
Faraday's predictive modeling eliminates subjectivity from lead scoring by incorporating both intuitive and non-intuitive data points that traditional lead scoring might miss. The model updates continuously with new customer data, ensuring that changes in the market or product offerings are reflected in the score. Unlike rule-based scoring, which can become outdated, predictive scoring always evaluates leads based on the most current and relevant information.
Additionally, Faraday's out-of-the-box identity graph eliminates the need to collect identity criteria or rely on expensive data vendors. With just an email or physical address, Faraday can instantly access over 1,500 data points on nearly every adult consumer in the country, both providing a deep well of data to find signals and eliminating the cold start problem of how to score leads with very little data.
The results
4x higher lead conversion
The marketing and analytics team at this nationwide home improvement brand integrated their lead data into the Faraday platform. The data used for training these models included multiple years of lead, appointment, and customer records. The Faraday platform automatically associated these records with thousands of demographic, property, financial, and lifestyle attributes found in the Faraday Identity Graph.
From there, the brand quickly built predictive lead scoring models to assess and rank leads based on their likelihood to convert into paying customers. The models found objective patterns across the client and Faraday's data that clearly distinguished a good lead from a bad lead.
To validate the accuracy and effectiveness of Faraday's lead scores, the home improvement brand engaged the agency Bold Orange to conduct a thorough analysis of the efficacy of the predictive lead scores, comparing Faraday model predictions against actual lead conversions and outcomes.
The analysis revealed that the conversion rate of leads in the top 10% of lead scores was 4x higher than the brand's baseline conversion rate, confirming the model's ability to accurately identify high-potential leads.
With this third-party verification in place, the brand deployed the models into production. The models scored the brand's leads in real-time the moment they entered their sales funnel and used the scores to prioritize leads within their call center. Now, the sales team has the confidence to know that they are talking to the best opportunity at any given moment.
Ready for easy AI?
Skip the ML struggle and focus on your downstream application. We have built-in sample data so you can get started without sharing yours.