Why explainable AI is so important for businesses
With AI being built into many businesses' workflows, concerns are rightfully being raised about its trustworthiness, and emphasis put on the importance of explainable AI.
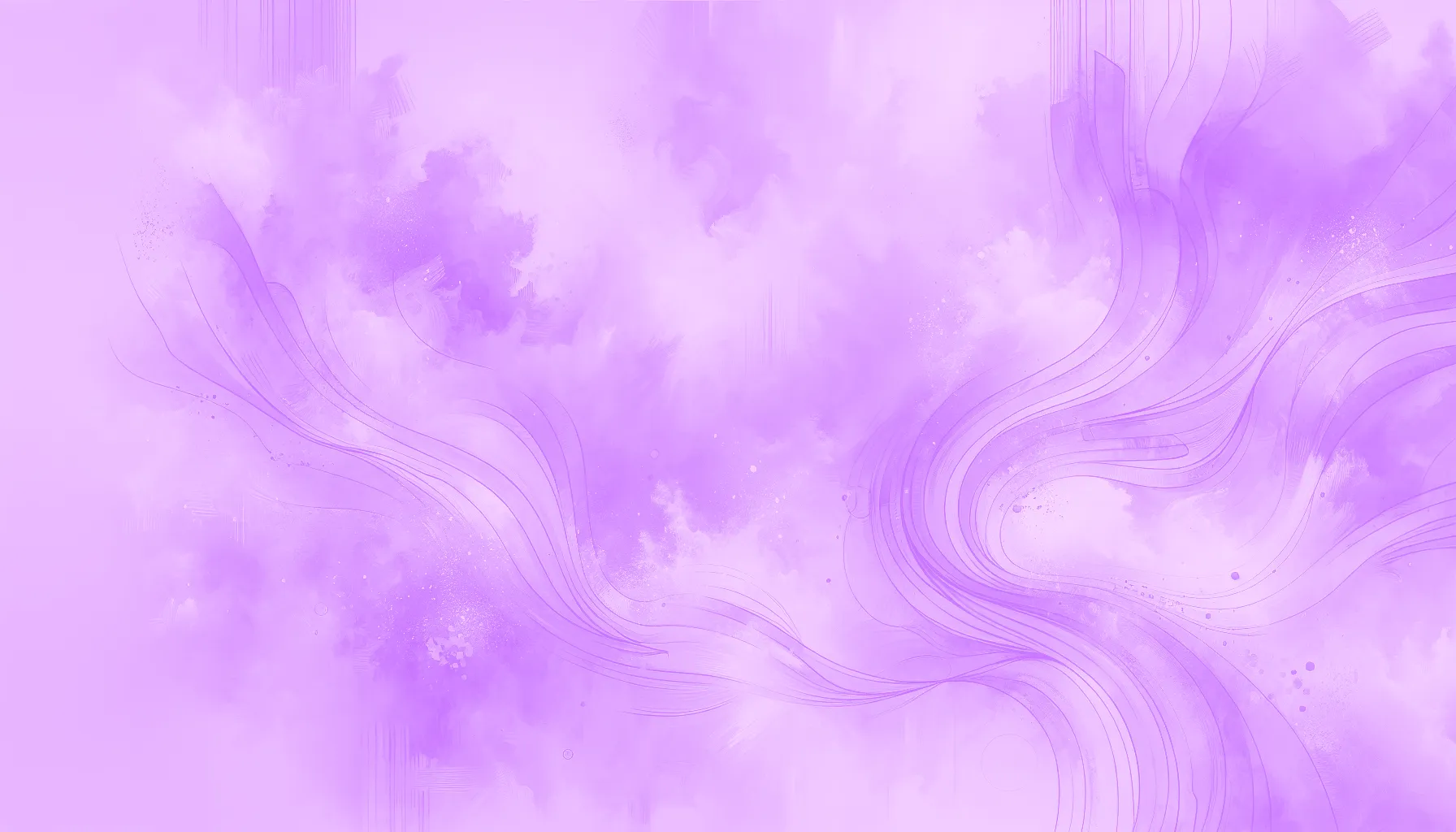
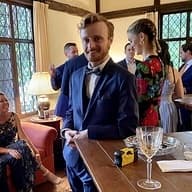
Regardless of what part of your business you find yourself in, your team has likely discussed implementing artificial intelligence (AI) of some sort in 2024–and it’s no wonder, with 33% of businesses already using AI and another 42% considering adopting it, the benefits of harnessing AI are too good to be ignored.
As leaders, employees, and consumers alike learn more about predictive models, machine learning algorithms, and various other pieces of the AI puzzle, questions naturally arise about how AI really works. Can the predictive models created be trusted? Can they produce biased results? Making complex machine learning algorithms and their results explainable to humans has proven to be a challenging feat for organizations to overcome in a tangible way, though there are significant benefits for those that do.
Put simply, if you’re going to change the way you interact with customers based on what AI tells you, how can you trust what it’s telling you?
What is explainable AI?
Historically, AI has been plagued by the black box problem, in which artificial intelligence reaches purportedly valuable conclusions—finding high-scoring leads, producing compelling generative content, and so on—and the user is left with the option to take it or leave it. No insight was provided into how the AI reached the conclusion it did, and what data it used to do so, thus the term “black box.”
Explainable AI aims to solve this problem by providing much-needed insight into the inner workings of predictive models so that the end-result of a predictive model offers both the sought-after conclusions, as well as how those conclusions were reached. This means that deploying customer predictions and other AI-generated content can be done with confidence, rather than a hand-wave.
Why does explainable AI matter?
According to IBM, 9 in 10 consumers say trust is the most important deciding factor when deciding which brand to adopt. People have never been more keenly aware of the importance of brand trust, and how quickly it can vanish. The need for consumers to trust brands they interact with has resulted in the need for explainable AI, and for the black box to be removed. IBM also reports that general consumer trust in businesses dropped from 48% in 2021 to 20% in 2023, meaning that businesses need to employ frameworks like explainability that help establish and maintain trust in the relationship for consumers to even consider making a purchase. The relationship between trust and explainability extends far beyond direct-to-consumer goods, too.
In finance—a space that has historically struggled with bias—AI can be used to determine whether or not an applicant should receive a loan, which leaves the door open for their machine learning algorithms to use historically biased data to permit or deny a loan. AI must be explainable in order for lenders, or anyone else building or employing it, to consider it for use.
Benefits of explainable AI
Explainable AI enables businesses that employ AI pipelines to properly interpret and understand its output without requiring data science degrees. This benefit is twofold: first, the importance of understanding the tools you’re working with rather than just taking their word for it can’t be overstated. Knowledge is power, after all. This leads straight into the second, in that it allows you to not only understand why the AI reached the conclusion it did, but then enables you to take that reasoning a step further by analyzing its context. If you’re using machine learning algorithms to find out which of your customers is most likely to churn, you can dig into its explainability to reveal what traits about them helped make that critical decision. Similarly, explainable AI can help reveal and mitigate bias in your data, which helps you stay compliant with local laws and regulations.
Additionally, a foundation of trust is built (and maintained) between the business and its customers, which is a rare commodity in 2024. With existing customers generating 72% of company revenue, a happy customer base that you’ve nurtured with trust will keep coming back for more–and they’ll refer their friends, too.
Challenges of explainable AI
With such impactful benefits on the table, why hasn’t explainable AI become mainstream? The simple answer is that it’s difficult. Entire classes of ML algorithms are fundamentally incapable of effectively explaining their inferences, so you have to choose your methodology carefully. Explainability also requires well-prepared and structured attributes that not every organization is lucky enough to have on the shelf. Finally, in a commercial environment where data science resources are both scarce and overburdened, AI explainability never quite seems to make it to the top of the todo list.
Explainability is built into Faraday
While businesses that are fully committed to building their own AI pipelines need to overcome the hurdles involved in implementing explainable AI, many businesses are instead adopting platforms like Faraday that are purpose-built for using AI–and in Faraday’s specific case, predicting customer behavior.
Faraday has long been a proponent of responsible, explainable AI. In fact, it’s one of our founding principles. Every predictive model that Faraday users create allows customization of what traits are used, allows removal of protected class data, and comes with a full report that details things like the most important traits that helped build the model. In 2023, we released score explainability, a feature that outlines each of the most influential pieces of data within an individual person’s predictions, and how much influence they had.
Let’s use the above graphic to visualize the churn prediction example in the previous section. Jane Madison has a 75% probability of churning, and the explanation provided in the predictive model that scored Jane said that the fact that Jane is a millennial played a major role in why she’s so likely to churn. Meanwhile, John’s children made it less likely that he would churn. Not only do we know that Jane is more likely to churn than John, but we know why.
In the end, explainable AI results in tangible benefits for both the businesses employing it and their customers. Businesses are able to understand their own tools, and use that understanding to reveal impactful pieces of insight like why exactly Jane Madison is going to churn. From a consumer perspective, businesses employing explainable AI stand out as trustworthy and worth interacting with–and worth referring their friends to do the same.
Ready for easy AI?
Skip the ML struggle and focus on your downstream application. We have built-in sample data so you can get started without sharing yours.