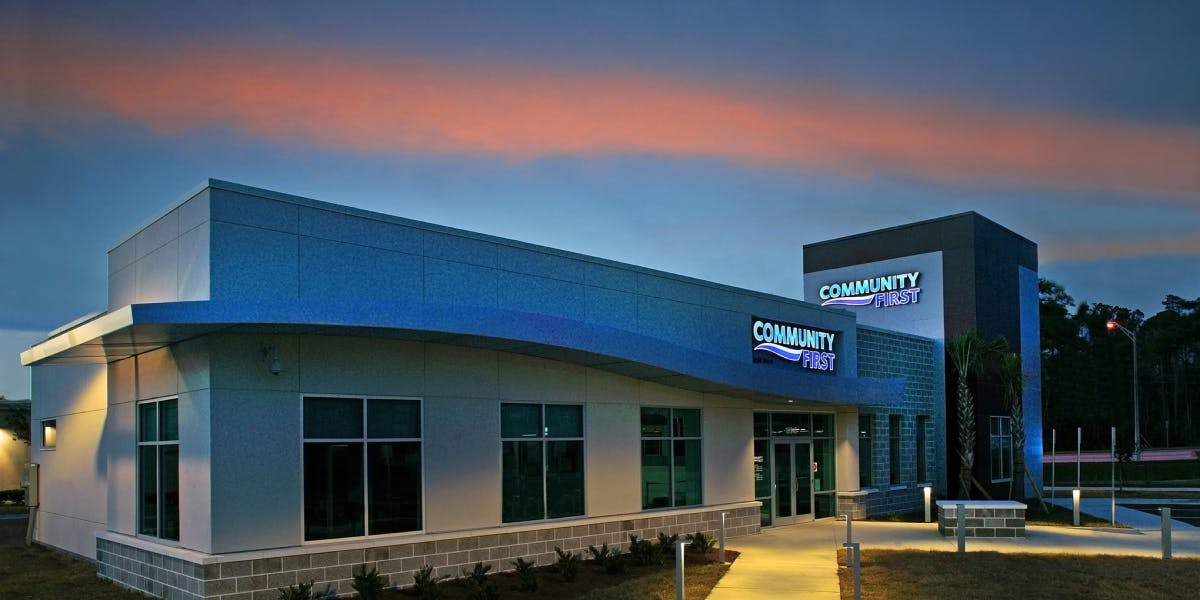
How Community First Credit Union aquired and engaged valuable credit union members with direct mailers
2 min read
Community First Credit Union (CFCU) has come a long way since opening their first office in a Jacksonville high school basement in 1935, and for good reason. Deeply invested in their local community, the credit union has grown into a billion-dollar business with 19 branches, serving over 135,000 members. They’ve always put their members first, tailoring products to fit individual needs and leveraging innovative technologies to create enjoyable banking experiences.
Outsmarting, not outspending the competition
CFCU knows that their member-first mindset alone isn’t always enough to compete with big bank budgets and agile fintech startups. They needed a better way to find great members and keep them engaged and happy in the long run. The credit union executed two direct mail campaigns as acquisition and engagement initiatives. To find out how well Faraday’s predictive audiences would perform, they were compared to those of traditional segmentation methods.
Putting predictions to the test
CFCU needed a cost-effective way to acquire members that would want to take advantage of a variety of their financial offerings. The credit union used the Faraday platform to predict which consumers were most likely to become their next loyal members. To test the model, CFCU ran a direct mail campaign with a 50/50 audience split (50% from high-scoring model predictions; 50% from traditional segmentation techniques). Compared to traditionally segmented audiences, the high-scoring prospects identified by Faraday’s model opened accounts with 61% higher account balances, 24% higher net interest revenue, and a 24% lift in projected ROI.