How this financial services provider optimized their direct mail outreach in just one month
In their first month with Faraday, this financial services provider used lead suppression to cut 80,000 low-value mailers and save nearly $40,000.
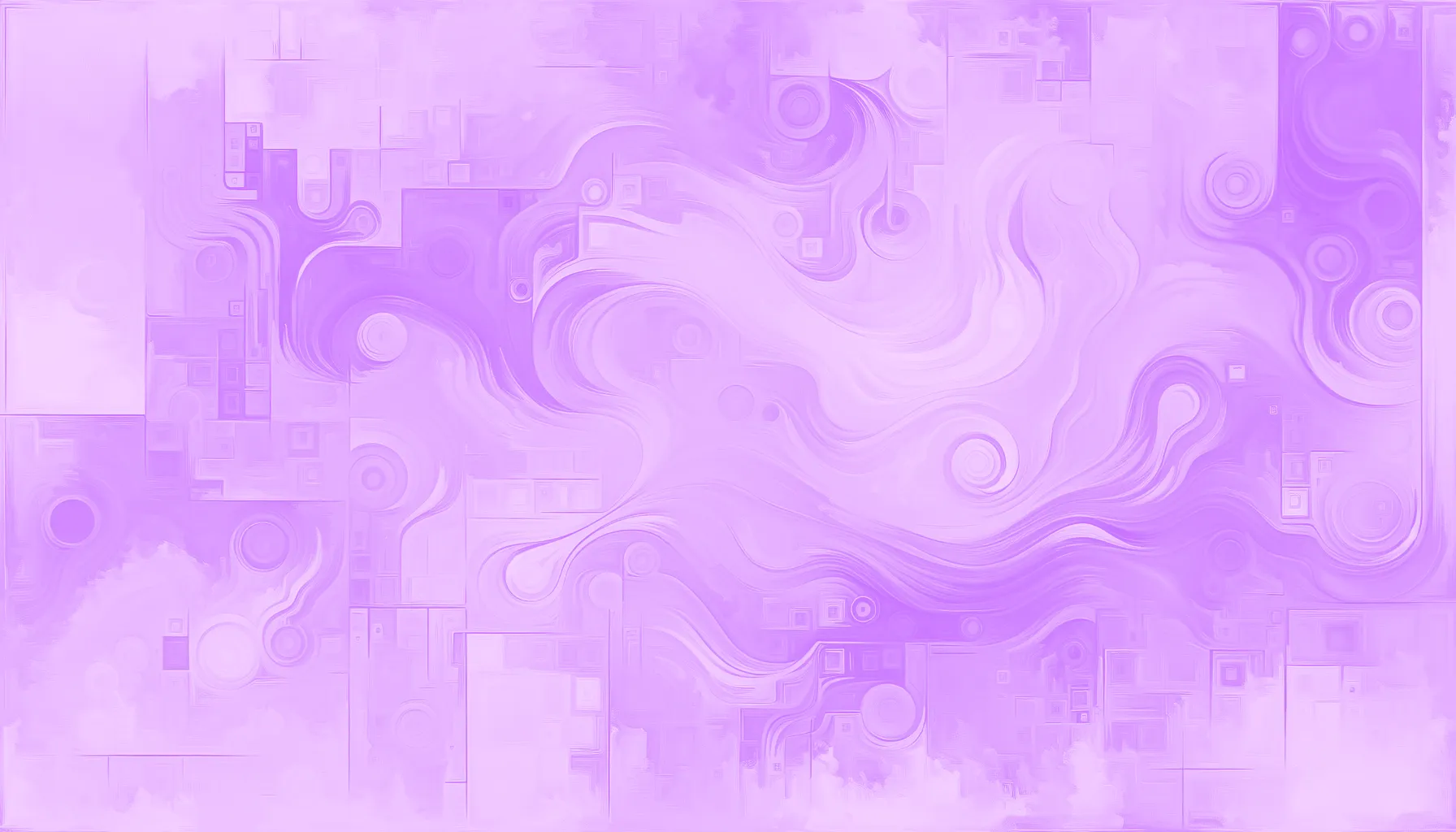
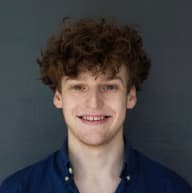
Direct mail remains a core acquisition strategy for many financial services providers, but it comes with a hefty price tag. Each mailer represents an investment, and when sent indiscriminately, costs can spiral with little return.
When this client first came to us at the end of 2024, like many in the industry, they relied primarily on instinct-based direct mail strategies, meaning a significant portion of their outreach was reaching consumers who were unlikely to convert. They knew they were overspending but lacked the tools to pinpoint which mailers were worth sending—and which could be cut without hurting revenue.
This client needed a solution that could optimize their outreach, reduce waste, and maintain strong enrollment numbers. Most of all, they wanted confidence in whatever decision they made—all without disrupting their existing workflow.
Lead suppression with Faraday
And that’s where Faraday came in. As soon as we identified their challenges, we quickly devised a targeted strategy to help.
The answer? Faraday’s lead suppression template.
To enact this strategy, we started by inputting the provider’s recent lead data into our system (maintaining rigorous data privacy standards like HIPAA, GDPR, and SOC-2 compliance throughout the process). This data was then enriched through our identity resolution process with 3rd party data from the built-in Faraday Identity Graph (FIG), which includes over 1,500 attributes on more than 240 million U.S. consumers and their households, and features nuanced demographic and behavioral indicators that influence consumer actions—ranging from property ownership to life events and purchasing habits.
From there, new predictive models were created and trained on this historical enrollment data (now enriched with 3rd party insights), identifying patterns and trends that distinguished high-value leads from low-value ones. Then, using advanced machine learning techniques, the system assigned each lead a predictive score, ranking them into ten distinct deciles based on their likelihood to convert. With this scoring system in place, a cut-off threshold was established to determine the point at which the cost of printing and sending a mailer outweighed the expected return, ensuring that outreach efforts remained cost-effective.
Finally, Faraday delivered an optimized, high-fit mailing list back to the provider and armed with this refined list, they were able to move forward with confidence, ensuring that every mailer sent had a greater likelihood of driving enrollment. For a visual breakdown of this process, check out our recent blog on lead suppression, here.
So how did it go?
The results (so far)
If you noted the timestamp on this article, you might be thinking to yourself, “Well, they reached out at the end of 2024 and this was published on Feb 21, 2025, so did they even get the models rolled out yet?”
Well, despite the short timeframe, they not only deployed the models and sent direct mail based on their predictions, but have also already seen a significant reduction in costs, all while maintaining strong engagement with high-quality leads.
Indeed, by suppressing low-likelihood recipients, they were already able to send over 80,000 fewer mailers, producing a net savings of nearly $40,000, demonstrating the immediate financial benefit of a more targeted approach.
These early financial wins were certainly affirming, but it had only been a month. How did we know that they were actually the result of our model and not just an uptick consumer interest this year?
Ongoing model validation
At Faraday, we take the validation of our models very seriously. We do this before predictions are deployed through our holdout testing process and continue testing on an ongoing basis even after successful implementation. In this case even though we were already seeing positive results, we wanted to see data that confirmed (or challenged) our assumptions. So we conducted further analysis on our models after we started getting results back to confirm that our predictive scoring was actually aligning with real-world outcomes.
And we found what we were looking for: Leads with the lowest predictive scores showed below-average conversion rates, validating the suppression strategy. Meanwhile, leads in the highest-scoring segments consistently outperformed the average, reinforcing the value of prioritizing the right audience. And what’s even better is that this correlation is only expected to strengthen as more data flows through the model, further refining predictions and maximizing efficiency.
While these financial results would be impressive on their own, they also highlight how quickly Faraday’s models can be deployed and optimized. The contract was signed in late-December, and within weeks, the provider was already seeing meaningful returns. This quick turn around, combined with the ability to continuously refine and improve targeting over time, positions them for even greater efficiency and cost savings in the months and years ahead.
Next steps
With direct mail suppression already demonstrating clear value, the provider is now looking to further optimize their customer outreach efforts. A key next step? Call center list scoring.
Similar to how Faraday’s predictive models helped refine direct mail targeting, they can also help prioritize leads for call center campaigns. By focusing call center agents’ efforts on high-likelihood prospects, the provider can reduce wasted calls, improve conversion rates, and increase overall campaign efficiency.
We can’t wait to see how they use this technology to generate even more value for their business!
In conclusion
In just a short time, this financial services provider has revolutionized its direct mail strategy, reducing unnecessary costs while maintaining strong engagement with high-value leads. Faraday’s predictive modeling has enabled them to move beyond broad, instinct-driven targeting to a data-backed approach, ensuring each mailer sent delivers greater value. And with tens of thousands of dollars saved in only a few weeks, the provider has already realized the immediate financial benefits of a more targeted outreach strategy.
What’s more, looking forward, the provider is well-positioned to refine their targeting approach even further. By continuously updating and optimizing their predictive models, and exploring additional use cases like call center list scoring, they can maximize the efficiency of all customer acquisition channels.
For this company, this is just the beginning of a smarter, more efficient approach to customer engagement—one that will continue to drive results well into the future.
If you'd like to know how Faraday can produce similar results for your business, sign up for free!
Ready for easy AI?
Skip the ML struggle and focus on your downstream application. We have built-in sample data so you can get started without sharing yours.